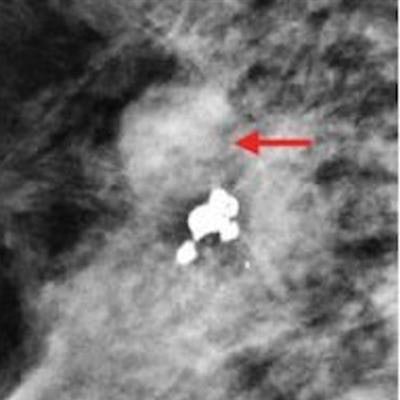
An artificial intelligence (AI) model trained with mammography images shows promise for identifying breast lesion subtypes and thus improving the effectiveness of biopsies, according to a study published October 25 in Radiology.
A group led by Vesna Barros, PhD, from IBM R&D Laboratories in Mount Carmel, Israel, found that their AI model was highly accurate in identifying lesion subtypes such as invasive lesions and ductal carcinoma in situ (DCIS) in breast images taken of Israeli and U.S. women.
"Our preliminary results are encouraging and show that our models have the potential to reduce biopsy sampling errors and decrease costs associated with false-positive findings," the team wrote.
AI continues to show its potential in radiology, including breast imaging, but previous studies have focused on smaller, retrospective data sets and patient populations consisting mostly of women with normal and cancer-free mammography results. In contrast, the study Barros and colleagues conducted was based on a screening population with mammography exams that led to biopsies; they included screening mammograms with visible findings that were later part of a diagnostic workup following abnormal screening results.
Along with cancer or noncancer classification, the researchers explored whether biopsied lesions detected at mammography could be further classified into more specific subtypes such as DCIS, invasive cancer, high-risk lesions, and benign lesions. The team also investigated whether including clinical characteristics from patients could improve the algorithm's accuracy.
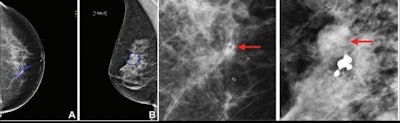
The algorithm models combined convolutional neural networks with supervised learning algorithms (that is, those trained using annotated images). They were trained to make breast lesion predictions with data from 2,120 Israeli women in Israel and U.S. 1,642 women; the Israeli model was validated with data from 456 women and tested with data from 441 women, while the U.S. model was validated with data from 350 women and tested with data from 344 women. Both models' performance was evaluated using the area under the receiver operating curve (AUC) measure.
For classifying pathologies, the best-performing algorithms achieved an AUC of 0.76 for DCIS, 0.85 for invasive carcinomas, and 0.82 for benign lesions, respectively. These included both clinical and imaging features in the Israeli training and test sets.
For predicting malignancy in the test sets, the algorithms obtained an AUC of 0.88 for Israeli women and 0.8 for U.S. women (p = 0.006).
The researchers emphasized that these results may not hold for other cohorts of patients and that generalizability across populations should be further studied.