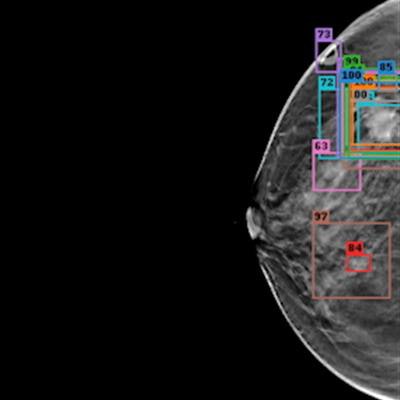
Artificial intelligence (AI) algorithms can deliver strong performance for detecting tumors on digital breast tomosynthesis (DBT) exams, according to results from an AI challenge published February 23 in JAMA Network Open.
A group led by Nicholas Konz from Duke University hosted a challenge where eight teams developed tumor detection algorithms using a provided dataset of over 22,000 DBT volumes. They found that teams were able to achieve high tumor detection performance on a challenging testing set of over 1,700 unseen DBT volumes.
"This vastly outperformed prior methods, because our challenge's competing participants had to develop cutting-edge novel approaches to solving this task," Konz told AuntMinnie.com. "By releasing the code of algorithms, our dataset, and an easy-to-use standardized public benchmark for anyone to test their own DBT tumor detection algorithms, we present a milestone for research in DBT tumor detection."
Previous research suggests that DBT improves cancer detection rates over conventional mammography, but it also adds reading time to radiologists' workloads. Konz and colleagues suggested that deep-learning algorithms could help lessen workloads while maintaining high-quality imaging.
However, they also pointed out that AI in medical imaging faces challenges stemming from a lack of sufficient, well-organized, and labeled training data, as well as a lack of benchmark and test data and clearly defined rules for comparing algorithms.
In hopes of providing a foundation for the future open development and evaluation of lesion detection algorithms for DBT, the researchers created a collection of analyses and resources for researchers based on a new publicly available data set.
To get the wheels rolling on this, the researchers hosted a challenge called DBTex, featuring eight teams from around the world. DBTex was divided into two phases: December 14, 2020 to January 25, 2021, and May 24 to July 26, 2021. The challenge task was the detection of masses and architectural distortions in DBT scans.
Challenge teams developed and trained their detection methods on a data set of healthy participants, with limited scans containing lesions. These came from a large, recently released, public, radiologist-labeled data set of 22,032 DBT images from 5,060 patients.
After the training phase, participants were provided with a smaller validation data, and at the end, teams applied their methods to a previously unseen test set of scans with normal and cancerous tissue for final rankings. Pathology and lesion locations of the training set were shared with participants as a reference standard, but they were made unavailable for the validation and test sets.
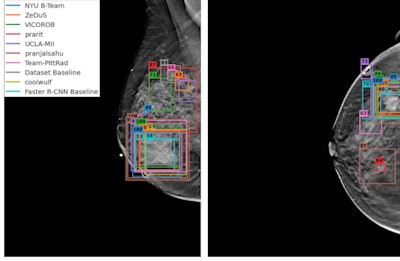
In the first phase of the study, teams were given 700 scans from the training set, 120 from the validation set, and 180 from the test set. The groups received the full data set in the second phase.
When the results were aggregated, the average sensitivity for all submitted algorithms was 87.9%. For teams that participated in the challenge's second phase, it was 92.6%. As for the groups themselves, NYU B-team based out of New York University-Langone Health won the challenge. Taking second was ZeDuS, based out of IBM Research-Haifa.
Performance of top-performing teams participating in DBTex | |||
Ranking | Team Name | Affiliations | Average sensitivity for biopsied lesions |
1 | NYU B-team | NYU-Langone Health | 95.7% |
2 | ZeDuS | IBM Research-Haifa | 92.6% |
3 | Vicorob | Vicorob-University of Girona | 88.6% |
4 | Prarit | Queen Mary University of London-CRST and School of Physics and Astronomy | 82.2% |
Konz said that the improved performance of the leading submitted algorithms seen in the challenge over baseline approaches was made possible by "the intense and competitive development of methodologies designed to handle these difficulties." He added that by providing a public standardized DBT tumor detection benchmark, radiologists can better interpret and compare the performance of tumor detection algorithms.
"This is especially important because AI research typically advances quickly, so new approaches may be developed regularly," he told AuntMinnie.com.
Konz also said that the group plans to host future phases of the tumor detection challenge for further detection algorithm research.
"We are also researching using AI to approach other clinically relevant medical image analysis tasks, such as anomaly detection and tumor segmentation," he added.