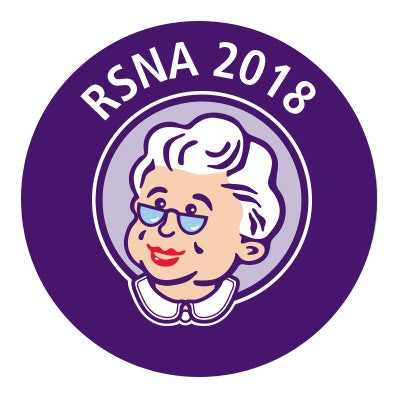
CHICAGO - Although they may yield impressive performances on carefully selected datasets, artificial intelligence (AI) algorithms need to be tested on real-world cases prior to being deployed in clinical practice, according to research presented on Tuesday at the RSNA meeting.
Researchers from Massachusetts General Hospital (MGH) compared the performance of their intracranial hemorrhage (ICH) detection algorithm on real-world cases with the initial dataset used to test the algorithm. Although they found that performance of the deep-learning model declined significantly on the real-world cases, they were able to vastly improve specificity after retraining the algorithm with a better balance of training cases.
"If we know the reality and develop AI accordingly, we are able to make AI models that can provide meaningful values in a clinical setting," said presenter Hyunkwang Lee, PhD.
After training a convolutional neural network (CNN) to detect ICH on noncontrast head CT scans, the MGH researchers found that it produced a high level of performance. They then wanted to assess how the system would perform in the real world.
Lee and colleagues gathered 2,606 consecutive cases of noncontrast head CT performed at their emergency department from September to November 2017. The cases were labeled as positive or negative for ICH using natural language processing (NLP) of clinical reports. Of the 2,606 cases, 163 were positive for intracranial hemorrhage, according to the NLP analysis.
Performance for detecting intracranial hemorrhage | ||
AI performance on test dataset | AI performance on real-world dataset | |
Sensitivity | 98% | 87.1% |
Specificity | 95% | 58.3% |
Area under the curve | 0.993 | 0.834 |
The researchers then delved further into data to find out why the model's performance dropped. A neuroradiologist with more than 20 years of experience reviewed 21 false-negative cases and found that eight did not contain acute bleeding (report hedging). Eleven cases contained small bleeding not visualized on axial CT images and two were small (3 mm and 10 mm) acute subdural hematomas, Lee said.
The 1,018 false-positive cases were split into five sets to be reviewed by five neuroradiologists. They found that the false-positive cases were caused by the following:
- Hyperdense falx or tentorium: 1,580 slices/420 cases
- CT artifacts (motion, streak, beam hardening, head tilt, etc.): 1,545 slices/463 cases
- Bleeding (chronic ICH, extracranial bleeding, hemorrhagic tumor): 875 slices/149 cases
- Calcification (encephalomalacia, meningioma, metastatic mass, vasogenic edema, postsurgical change, old infarct): 348 slices/130 cases
- Other (dense blood vessels, deep sulcus, subdural hygroma): 743 slices/373 cases
The researchers also observed that the testing dataset and the real-world dataset contained different numbers of certain types of cases generated by scanners from two vendors.
In light of these findings, they added 674 false-positive cases (3,153 slices) with a better balance between those two vendors to the previous development set in order to train a new model. The test set now included 817 cases.
The new training data led to a significant improvement in specificity, he said.
"By understanding the nature of incorrect prediction in the real-world data, the model can be improved through constant feedback from expert radiologists, facilitating the adoption of such tools in clinical practice," Lee said.
Future work
The researchers are now planning to validate the diagnosis assigned via the NLP of clinical reports. They also would like to improve the model by retraining the CNNs to distinguish between chronic, subacute, and acute bleeding and to recognize other pathologies, Lee said.
In addition, they want to validate the improved model in different settings, including using different CT manufacturers, image acquisition/reconstruction protocols, and patient populations.