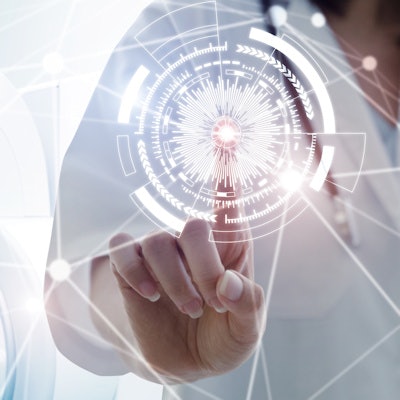
What if you took two of the most exciting technologies in medical imaging and put them together? That's the promise behind the application of artificial intelligence (AI) to digital breast tomosynthesis (DBT). The upcoming RSNA 2019 meeting will offer an excellent look at the progress being made in integrating AI with DBT.
While AI is being applied to various imaging modalities, it appears to be a perfect match for DBT. Also known as 3D mammography, DBT offers a number of improvements over traditional 2D full-field digital mammography (FFDM) in terms of cancer detection, but it also presents unique challenges that lend themselves well to AI, such as higher radiation dose and longer interpretation times.
At next month's RSNA 2019, numerous research presentations will explore ways that AI can make DBT better, either via improved efficacy or better patient safety.
Bullish on AI
One researcher who is bullish on AI's potential in DBT is Dr. Emily Conant of the University of Pennsylvania in Philadelphia.
"The whole field of AI is blooming," Conant said.
Conant led a team that earlier this year showed that using AI software to interpret DBT exams cuts reading time and improves breast cancer detection, sensitivity, and specificity. At RSNA 2019, Conant will present a subanalysis of that data (Sunday, December 1 | 11:45 a.m.-11:55 a.m. | SSA01-07 | Room S406A).
In her RSNA talk, Conant will discuss how the use of an AI algorithm with DBT improved sensitivity, specificity, and reading time, whether interpreting DBT images with conventional 2D digital mammography or DBT and synthesized 2D mammography.
More specifically, Conant's group found that AI boosted area under the curve (AUC) from 0.781 to 0.848 for DBT plus conventional 2D mammography and from 0.812 to 0.846 for DBT with synthesized mammography. Reading times improved 55.1% for using AI with DBT and conventional 2D mammography and 44.4% for DBT with synthesized mammography.
In another talk at RSNA 2019, a convolutional neural network (CNN) that uses 2D and 3D mammograms simultaneously will be presented by a team that includes Dr. Xiaoqin Jennifer Wang and Nathan Jacobs, PhD, of the University of Kentucky (Tuesday, December 3 | 3:00 p.m.-3:10 p.m. | SSJ02-01 | Room E451B).
Their research highlights the potential of using 2D and 3D mammography together to detect cancer by virtually slicing through 3D DBT images and comparing the slice difference with 2D mammograms, Wang explained.
"We developed a neural network architecture that embodies elements of what the radiologists do in clinical practice," Jacobs said. "You have higher spatial resolution with the 2D data. If you are working with the 3D data, there may be smaller features in the scan that are hard to determine purely from 3D."
In their study, AI analysis of combined 2D and 3D mammography data produced an AUC of 0.93 for differentiating cancer from benign lesions, a performance better than 2D or 3D mammography alone (AUC = 0.72 for 2D and 0.66 for DBT).
Jacobs noted they will continue to work on increasing the accuracy of the model.
"We will explore technical issues of confidence in the neural network," he said. "We do not want it to say 100% benign and have it wrong."
Still another team of investigators will show at RSNA 2019 results from their study of 5,000 women who presented for screening DBT between 2013 and 2017, with this dataset having biopsy-proven results. Researchers used deep learning to see if the technology would reduce DBT interpretation time, finding that it was able to filter 37% of normal exams, with 97% sensitivity. The findings suggest that AI can decrease the interpretation workload of radiologists (Sunday, December 1 | 10:45 a.m.-10:55 a.m. | SSA01-01 | Room S406A).
Another investigation out of South Korea explored using a deep-learning tool to analyze large mammography datasets. Calling their technology a data-driven imaging biomarker in mammography (DIB-MMG), the tool generates a heat map that indicates malignancy (Sunday, December 1 | 11:35 a.m.-11:45 a.m. | SSA01-06 | Room S406A).
But despite the promise of the research, more investigation is required, said Hyo-Eun Kim, PhD, head of the breast radiology division of AI software company Lunit in Seoul.
Before the tool can be applied to clinical practice, "more validation and study are needed," Kim noted.
Tackling radiation dose
One of the most serious challenges with DBT has been radiation dose. Breast imagers have found 3D mammography to be tremendously useful for detecting cancer, but many do not want to part with the 2D views they've come to rely on from traditional mammography. Performing another exam to provide those views boosts radiation dose to patients -- a major concern in any screening environment.
"Repeated DBT for annual screening would increase cumulative radiation exposure and lifetime attributable risks for radiation-induced cancer," explained Kenji Suzuki, PhD, formerly of the Illinois Institute of Technology and now a professor at the Institute of Innovative Research at the Tokyo Institute of Technology in Japan.
Synthesized 2D mammography is a possible solution by enabling 2D-like images to be reconstructed from the slices acquired with a 3D exam, cutting dose by 50%. But how well does AI perform when examining synthesized 2D data?
To find out, Suzuki and colleagues developed a CNN that demands a very small number of training cases. At RSNA 2018, they presented data showing that the algorithm reduces dose in DBT exams by 82%.
"Substantial radiation dose reduction by our technique would benefit patients by reducing the risk of radiation-induced cancer from DBT screening," Suzuki said.
The way forward
Going forward, AI has the potential to make a great technology -- DBT -- even better. But as with the use of AI for other applications, much depends on the availability of real-world exams to develop and test new algorithms. It's an especially relevant conundrum with DBT, given the relative novelty of the technology.
"We have had databases of mammography for a long time, but in terms of really strong databases with outcomes and annotated images in tomo, there are not as many," said Conant, noting that DBT has been in use for less than a decade versus mammography, which has been in use for decades.
"We need to make those huge databases of very diverse patient populations that take into account ethnic diversity and breast type diversity," she said. "We need to get better at quantitating breast density and the complexity and texture of the breasts."
With AI research endeavors using datasets from different sources, Conant encourages careful discrimination of the conclusions reached in AI research -- even studies presented at RSNA.
"When you read the tomo AI papers, you have to look and see if they are using the 2D synthetic or 2D with tomo or on the tomo slices," she stressed. "Not a lot of [research] groups are doing it on the tomo slices."
Still another area that could stand improvement in AI research is image acquisition, according to Conant.
"We need to improve the image acquisition and presentation of the image and the data in a more refined, coalesced way, being able to compare that over time, in a reproducible way that will help in patient care," she said.
A major challenge that lies ahead is to determine if AI algorithms will apply to images acquired by systems from multiple vendors.
"How translatable are these algorithms across different vendors?" Conant asked. "How reproducible and robust are the results?"
Conant pointed out that her research has suggested that gains are to be had with AI in DBT through reducing reading time, but she cautioned that the results of what occurred "in the lab" may not automatically translate to a busy, clinical practice.
"With tomo, people worry about all the data and the time it takes to read it," Conant said. "It has been estimated that it takes two times as long [to read] as a 2D mammogram."
Paving the way to personalized medicine
Conant sees the future of AI and DBT in the integration of tools such as radiogenomics to pave the way for personalized medicine in cancer treatment, identifying the responses to variables like recurrence and the need for more treatment.
"If the lesion is upgraded to a high-risk lesion, we may want to incorporate patient data and genetic data," Conant explained. "If the patient has cancer, we would want to know her prognosis and how she responds to therapy. It will not be a one-size-fits-all approach. We will leverage all the data we can and use that as we go forward."
Obtaining responses to questions about risk and breast density is something where AI can definitely meet a need, Conant believes. "AI can do that more robustly than we do," she said.
And personalized medicine may ultimately prove to save costs, a particularly relevant concern with any screening exam, Conant believes.
"We can refine [our approach], so that everything does not need supplemental screening," she said.
In Conant's view, the "win-win" in AI with DBT will be a win for the patient, through improved breast cancer detection, and a win for the radiologist, allowing for greater productivity through things such as decreased reading time, freeing up time for more complicated cases.
"One of the questions is how many [scans] can AI read and never make a mistake?" Conant asked. "If the [AI] programs are able to predict that nothing will be missed, that is really exciting for radiology."