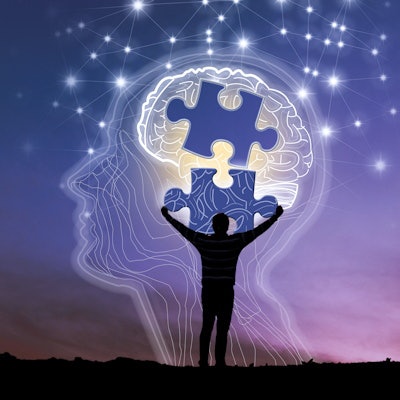
Artificial intelligence (AI)-based assessment of tau PET imaging data could help clinicians diagnose Alzheimer's disease, according to a study published April 29 in BioMedical Engineering Online.
A team at Kangwon National University in Chuncheon, South Korea, developed and tested machine-learning models for classifying stages of Alzheimer's disease using details from patient F-18 flortaucipir PET images. The algorithms performed well and could be helpful for clinicians diagnosing the disease, suggested first author Dr. Sang Won Park and colleagues.
"A more substantial application of this technology can be for determining whether a [cognitively unimpaired] patient will be classified with [mild cognitive impairment]," the group noted.
PET scans play a pivotal role in staging people with Alzheimer's disease based on visualizing radiotracer uptake by tau protein brain deposits. These deposits are thought to be involved in neurodegeneration associated with the disease. Based on the pattern of uptake, Alzheimer's dementia can be classified into three stages: cognitive unimpaired, mild cognitive impairment, and Alzheimer's dementia, the authors explained.
In this study, the group aimed to highlight the importance of this imaging data in Alzheimer's disease by using it as the basis for AI models that could potentially be used to stage patients.
The researchers culled 199 F-18 flortaucipir (Tauvid, Avid Radiopharmaceuticals) PET images from patients previously enrolled in a clinical trial by the Alzheimer's Disease Neuroimaging Initiative, a large international research project established in 2004. They extracted clinical variables and standard uptake value ratios (SUVR) from baseline scans of 74 cognitively unimpaired, 69 mildly cognitively impaired, and 56 patients with dementia and then developed different types of algorithms to predict these stages.
In the task of classifying between mildly cognitively impaired individuals and those with Alzheimer's dementia, a support vector machine model using only tau SUVR achieved an area under the receiver operating characteristic curve (AUC) of 0.88 (p < 0.05), which was the highest compared with other models.
In classifying between mildly cognitively impaired and cognitively unimpaired individuals, the AUCs of each classification model was also higher with tau SUVR variables than with clinical variables independently, which yielded an AUC of 0.75, according to the findings.
In addition, all of the machine-learning algorithms performed well using clinical and tau SUVR data for classifying between cognitively unimpaired and dementia patients, with a mean AUC > 0.96, the group reported.
Ultimately, the study confirms that the independent use of tau SUVR has a remarkable performance for classifying stages of Alzheimer's disease and that the metric may be useful in developing AI models to help diagnose the disease, the researchers noted.
"In this study, we demonstrated that the tau deposition level is very effective in classifying Alzheimer's disease stages when used together with clinical information that can be easily obtained at clinical screening," the group concluded.