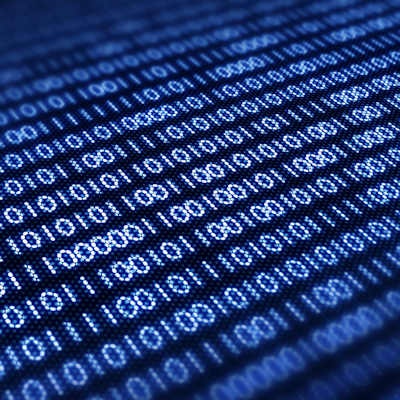
PHILADELPHIA - Artificial intelligence (AI) software can accurately predict a patient's gender on PET/CT scans, a capability that could help to avoid patient identification errors in PACS, according to research presented on Saturday at the Society of Nuclear Medicine and Molecular Imaging (SNMMI) annual meeting.
Researchers from Hokkaido University in Sapporo, Japan, trained a convolutional neural network (CNN) that produced an overall accuracy of 97% for predicting gender based on an analysis of the patient's PET/CT scan.
"The system might prevent patient misidentification," said presenter Keisuke Kawauchi.
Patient misidentification
Patient misidentification, such as when a patient is scanned and the images are registered to another patient on the PACS software, can sometimes lead to severe consequences. However, the correct matching of the image to the patient is hardly ever checked in clinical settings, Kawauchi said.
Misidentification errors could be prevented if a system could automatically predict patient characteristics such as sex, age, and body weight from the image itself and then generate alerts when it detects a mismatch, he said. Given the growing popularity of CNNs for image analysis tasks, the researchers sought to develop a CNN as an automated system for predicting the patient's gender from his or her FDG-PET/CT images.
The researchers gathered data from 6,435 patients -- including 3,653 men -- with an average age of 64.7 (range: 24-82 years) who had received whole-body FDG-PET/CT studies at their institution between January 2015 and August 2017. Patient gender was determined based on DICOM image tags. The PET/CT studies were acquired on a Biograph 64 (Siemens Healthineers) or a Gemini TF 64 (Philips Healthcare) scanner.
Maximum intensity projection (MIP) images (with a matrix size of 168 x 168 or 144 x 144) were generated on each scanner's workstation at various angles. The CT images in the study were used only for attenuation correction and not for classification purposes in this project, Kawauchi said.
The researchers chose to use the PET images for training the CNN because PET images are smaller than CT images, he said. MIP images were used because they always contain information on patient gender.
"Using MIP of PET, we suppressed the learning costs to reduce computational time," Kawauchi said.
Different training/testing approaches
Because two different scanners with different imaging and reconstruction techniques were used in the study, the researchers utilized three different training and testing approaches for their deep-learning initiative.
In the first experiment, images from both scanners were used to train and test the CNN. The second experiment involved training the CNN using images from the Biograph scanner and testing it on images from the Gemini scanner. Finally, the third experiment used Gemini images for training and Biograph images for testing. The original images from both scanners were resized to 64 x 64 prior to being input into the CNN.
Of the images in the study, 70% were randomly selected for training and the remaining 30% were set aside for testing. The training and testing process was repeated five times to calculate system accuracy.
The researchers found that the CNN produced the best accuracy when it was trained and tested using both sets of images.
Accuracy of CNN for predicting patient gender | |||
CNN trained on images from Gemini and tested on images from Biograph | CNN trained on images from Biograph and tested on images from Gemini | CNN trained and tested on images from both Biograph and Gemini | |
Accuracy | 81% | 89% | 97% |
"Therefore, it may be an effective method for multicenter studies," Kawauchi said.
He shared two cases in which the AI software incorrectly predicted the patient's gender. The first case involved a very slim male patient who was incorrectly predicted to be female.
"Females [tend to be] slimmer than males in Japan, so he could have been confused for female," he said.
The second case was of a relatively obese woman with head and neck cancer -- a male-dominant disease, according to Kawauchi. The software incorrectly predicted that the patient was a male.
Patient misidentification could be further prevented by predicting the patient's age and weight, as well as by comparing previous images of the patient, he noted.