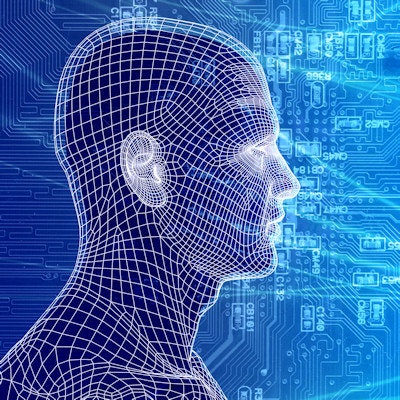
PHILADELPHIA - Artificial intelligence (AI) algorithms can help predict disease-free survival in patients with soft-tissue sarcoma, according to research presented on Sunday at the Society of Nuclear Medicine and Molecular Imaging (SNMMI) annual meeting.
Researchers from the University of Wisconsin School of Medicine and Public Health trained several machine-learning algorithms using a variety of different classifiers and found that the best-performing classifiers showed a promising level of accuracy for predicting clinical outcomes in soft-tissue sarcoma patients.
"Our results provide the potential clinical utility of machine-learning algorithms to improve the use of PET radiomics features to predict clinical outcome," said presenter Jihyun Kim, also of Asan Medical School in Seoul, South Korea.
Data analysis need
FDG-PET/CT can be an effective tool for identifying high-risk patients with soft-tissue sarcoma; previous studies have found the modality to be predictive of patient outcomes, Kim said. She noted, though, that recent advances in radiomics provide a large amount of quantitative features from medical imaging that require high-throughput data analysis.
"In order to assess patient outcomes from many imaging features, appropriate methods should be applied," she said. "Machine learning can be a potential solution to analyze and data-mine large radiomics datasets."
The researchers sought to investigate if machine-learning algorithms could improve the accuracy of survival predictions based on radiomics features from baseline FDG-PET and MRI studies of soft-tissue sarcoma patients. They first obtained clinical and imaging data from the U.S. National Institutes of Health's The Cancer Imaging Archive (TCIA) Soft-Tissue Sarcoma database, which included 48 patients with primary soft-tissue sarcoma. All patients had pretreatment FDG-PET/CT and MRI scans.
A volume of interest containing soft-tissue sarcoma was obtained from each patient's PET/CT images, and conventional tumor threshold methods based on standardized uptake values (SUV) -- SUV2.0 and SUVmax30% -- as well as a novel segmentation method -- MO-PET -- were calculated. The TCIA dataset also included manual contours of MR images on T2-weighted fat-saturated images. The researchers considered both conventional PET SUV-based features -- SUVmax, SUVpeak, SUVmean, metabolic tumor volume (MTV), and total lesion glycolysis (TLG) -- as well as 36 PET- and MRI-based texture features. Of these 36 PET- and MRI-based texture features, five were related to histograms, 20 were from gray-level co-occurrence, and 11 were from gray-level run-length matrices.
Machine-learning algorithm
The researchers evaluated six classification methods for the algorithm: naive Bayes, k-nearest neighbors, random forest, conditional random forest, tree-augmented naive Bayes, and logistic regression. The modeling performance was determined using tenfold validation, replicated 10 times, Kim said.
For the purposes of the study, the end point was disease-free survival from the diagnosis of cancer to progression, recurrence, or death. The researchers assessed accuracy by performing receiver operating characteristic analysis to define the area under the curve (AUC). Of the six classification methods tested for the algorithm, the tree-augmented naive Bayes and random forest classifiers performed the best.
Performance of 2 best machine-learning algorithms by PET segmentation method | ||
AUC for tree-augmented naive Bayes | AUC for random-forest classifier | |
SUV2.0 | 0.85 | 0.84 |
SUVmax30% | 0.81 | 0.76 |
MO-PET | 0.79 | 0.82 |
"We identified that machine-learning algorithm [tree-augmented naive Bayes] and [random-forest] methods had the highest prognostic performance to predict [disease-free survival] in [soft-tissue sarcoma] patients and improved the prediction value of PET features at baseline FDG-PET images."
These results now need further verification on datasets with larger numbers of soft-tissue sarcoma patients, according to the researchers.