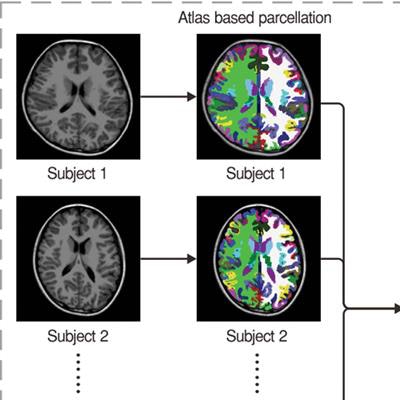
Researchers have developed a unique combination of radiomics, machine learning, and MRI brain scans to identify children and young teens with attention deficit hyperactivity disorder (ADHD) and differentiate between three types of the condition, according to a study published online November 22 in Radiology.
The data-gathering technique uses cerebral radiomic features, along with gray-matter and diffusion measurements from MR images, to distinguish between patients with ADHD and healthy control subjects, as well as between those with different ADHD subtypes (Radiology, November 22, 2017).
"In our study, we established an analysis framework on the basis of cerebral radiomics and machine-learning methods for ADHD diagnosis and subtyping," wrote lead author Huaiqiang Sun, PhD, and colleagues from West China Hospital of Sichuan University in Chengdu, China. "By identifying features relevant for diagnosis and subtyping, these findings may advance the understanding of neurodevelopmental alterations related to ADHD."
ADHD anomalies
ADHD is characterized by periods of inattention, hyperactivity, and impulsive behavior. There are three primary subtypes of ADHD:
- Predominantly inattentive
- Predominantly hyperactive/impulsive
- A combination of inattentive and hyperactive
Between 5% and 7% of children and adolescents worldwide are affected by the disorder, according to the ADHD Institute.
Currently, ADHD is diagnosed through observed and reported symptoms and psychoradiology. In recent years, MRI has been used to analyze the brain and explore connections between brain abnormalities and mental health and neurological conditions.
Sun and colleagues have taken the research one step further by creating an analytical framework for psychoradiology that includes cerebral radiomics, which extracts large amounts of quantitative information from MR images to identify ADHD characteristics.
The researchers consecutively studied 83 children between the ages of 7 and 14 years who were newly diagnosed and never treated for ADHD. The subjects had inattentive ADHD and combined subtypes. In addition, 87 healthy, similarly aged children were enrolled for comparative purposes. All subjects underwent MRI exams on a 3-tesla scanner (Magnetom Trio, Siemens Healthineers).
The newly developed radiomics model was then used to screen relevant signatures from more than 3,100 quantitative features taken from gray and white matter in the brain.
Radiomics discoveries
Sun and colleagues found no overall difference between the subjects with ADHD and the controls in terms of total brain volume or total gray- and white-matter volumes (p = 0.051). However, they did detect differences in specific regions within the brain. More specifically, alterations in the left temporal lobe, bilateral cuneus, and areas around the left central sulcus contributed significantly to distinguishing those with ADHD from typically developing controls.
Sensitivity for discriminating between patients with ADHD and the control subjects was 70.2% (± 7.1%), while specificity was 77% (± 5.8%) and mean accuracy was 73.7% (± 5.2%). Mean accuracy for differentiating between the ADHD inattentive and ADHD combined subtypes was 80.1% (± 6.2%).
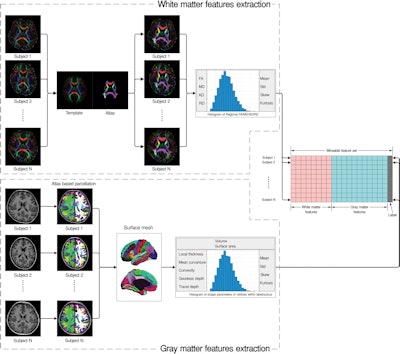
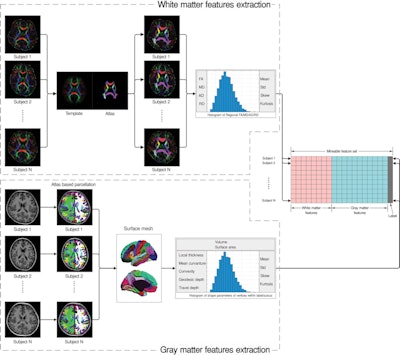
The diagram shows the workflow for extracting radiomic features from T1-weighted MR and diffusion-tensor images. FA = fractional anisotropy, MD = mean diffusivity, AD = axial diffusivity, and RD = radial diffusivity. Image courtesy of RSNA.
"This imaging-based classification model could be an objective adjunct to facilitate better clinical decision-making," said study co-author Dr. Qiyong Gong, PhD, in a statement. "Additionally, the present study adds to the developing field of psychoradiology, which seems primed to play a major clinical role in guiding diagnostic and treatment planning decisions in patients with psychiatric disorders."
The researchers plan to recruit more newly diagnosed ADHD patients to validate their results and learn more about imaging-based classification. They also plan to apply the analytic approach to other disorders and test its feasibility in a clinical environment, where the fully automatic analytic framework can be readily deployed, Gong said.