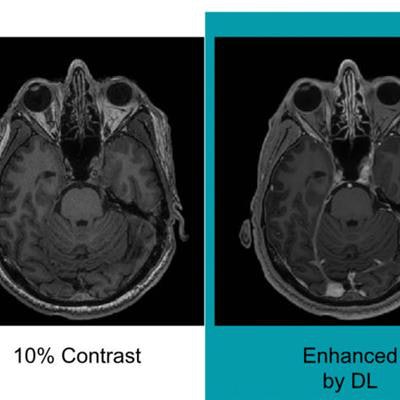
An artificial intelligence (AI) algorithm could enable diagnostic-quality MR images to be produced using a fraction of the typical dose of a gadolinium-based contrast agent (GBCA), according to research presented on Monday at RSNA 2018 in Chicago.
Researchers from Stanford University trained a deep-learning algorithm to generate synthesized MR images that approximate full-dose, contrast-enhanced MRI studies by analyzing precontrast and postcontrast acquisitions at 10% of the standard GBCA dose. In testing, these synthesized full-dose images were deemed to be comparable in image quality and contrast enhancement to those produced with a full dose of contrast.
Minute traces of gadolinium have been found to linger in brain tissue, bone, skin, and the liver long after administration of a GBCA. While proponents assert that the benefits of GBCAs outweigh their risks, a growing number of people are experiencing illnesses and debilitating symptoms that they attribute to gadolinium-based contrast. In the meantime, clinicians have been cautious about their use of GBCAs and how much dose they administer.
"There is concrete evidence that gadolinium deposits in the brain and body," said lead author Enhao Gong, PhD, in a statement. "While the implications of this are unclear, mitigating potential patient risks while maximizing the clinical value of the MRI exams is imperative."
For the study, the researchers used contrast-enhanced MRI exams performed for a variety of indications in 200 patients. They trained the deep convolutional neural network using three sets of MRI images: precontrast scans, a low-dose set acquired with 10% of the standard gadolinium dose, and a third set derived from scans with full 100% GBCA dose with 3D T1 inversion-recovery fast spoiled GRASS (IR-FSPGR) sequences. By doing so, the algorithm learned how to approximate and differentiate full-dose GBCA-enhanced scans from the zero-dose and low-dose images.
Neuroradiologists then evaluated the images based on contrast enhancement and overall diagnostic quality. They found that image quality did not differ significantly between the synthetic full-dose images produced by the deep-learning algorithm and the actual full-dose images. What's more, the results showed the potential for creating the equivalent of full-dose images without using a contrast agent, according to the researchers.
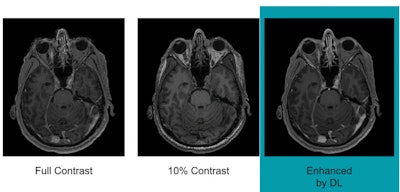
"With a large dataset, we demonstrated the deep-learning solution can generalize well, achieving robust and significant quality improvement over the low-dose contrast-enhanced MRI, using 10% or even less gadolinium dosage," the researchers concluded. "Low-dose gadolinium images yield significant untapped clinically useful information that is accessible now by using deep learning and AI."
Future research will include the evaluation of the algorithm in the clinical setting, across a broader range of MRI scanners and with different types of contrast agents.
"We're not trying to replace existing imaging technology," Gong added. "We're trying to improve it and generate more value from the existing information while looking out for the safety of our patients."
Gong received the RSNA's Trainee Research Prize - Resident for this research.