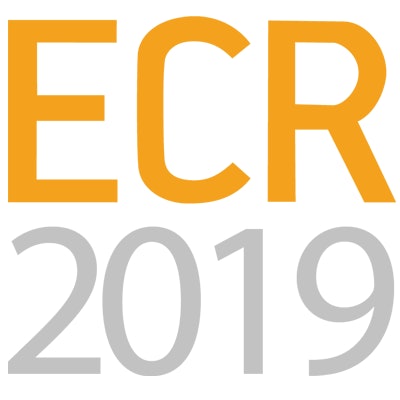
VIENNA - A newly developed deep-learning algorithm has shown promising results in the automated diagnosis of several neurological diseases based on routine MRI scans, according to a study presented on Thursday at ECR 2019.
Thus far, the algorithm has achieved high diagnostic accuracy in distinguishing normal MR brain images from scans of patients with brain tumors, ischemic cerebrovascular disease, or multiple sclerosis, based on initial T1- or T2-weighted sequences.
"Because our study is based on only T1- and T2-weighted images, after these scans, the clinician can know which disease it may be," said Dr. Yaou Liu, PhD, from the department of radiology at Beijing Tiantan Hospital. "They can select the optimum [follow-up] MRI protocol, whether to give contrast, 3D [fluid-attenuated inversion-recovery (FLAIR)], or diffusion-weighted imaging if stroke is suspected."
Patient workflow
Beijing Tiantan Hospital has one 1.5-tesla and six 3-tesla clinical MRI scanners, as well as one 7-tesla and one 3-tesla MRI device for research purposes. Given its nine MRI systems, the facility performs a high volume of MR imaging.
"That is why we want to have deep-learning methods to make our workflow more efficient," Liu said. "In the medical area, most deep-learning methods are used in diagnostic imaging, so our radiologists have a practical relationship with artificial intelligence."
The objective of this project was to identify abnormal MR images by automated methods to improve workflow efficiency. The hospital has many normal MRI results, so this type of deep-learning algorithm could help save time for radiologists, Liu added.
The second objective was to help clinicians select the optimal MRI protocol for different neurological diseases after they are detected by the algorithm. For example, should a patient receive contrast with an MRI scan for a suspected brain tumor? Is 3D FLAIR the best option to assess for multiple sclerosis?
From those decisions comes the researchers' third objective -- to direct patients to the correct clinical department for appropriate treatment.
Training and testing
To develop the deep-learning algorithm, Liu and colleagues randomly selected 5,806 MR brain images. That sample consisted of 1,064 normal images, 3,658 tumor images, 556 images with ischemic cerebrovascular disease, and 528 images from patients with multiple sclerosis.
The researchers chose those three diseases "because they are the most common cases in our hospital, and all the different diseases have very different treatments," Liu told ECR attendees.
The researchers then took 80% of the MR images from each category to compile a total of 4,639 images for the training dataset. The remaining 20% -- 1,167 MR images -- were used to test the algorithm.
The automated diagnostic method achieved sensitivity of 85%, specificity of 96%, and overall diagnostic accuracy of 94%, the group found.
Sensitivity by category was 91% for normal images, 90% for tumors, 84% for ischemic cerebrovascular disease, and 76% for multiple sclerosis. Specificity was 91% for normal images, 94% for tumors, 98% for ischemic cerebrovascular disease, and 99% for multiple sclerosis.
A few glitches
The deep-learning algorithm was not perfect, however; it did list 63 brain tumors as normal. In addition, 18 normal MR images were incorrectly diagnosed as brain tumors (8), ischemic cerebrovascular disease (5), and multiple sclerosis (5).
Liu acknowledged that the misidentifications are an early limitation of the algorithm, but he noted that radiologists will review the results to confirm or correct the initial automated diagnosis.
The researchers plan to further validate and simplify the algorithm with follow-up multicenter and longitudinal studies.
"We also need to extend [the algorithm] to other disease categories, like Alzheimer's, Parkinson's, and other neurodegenerative disease," Liu said.