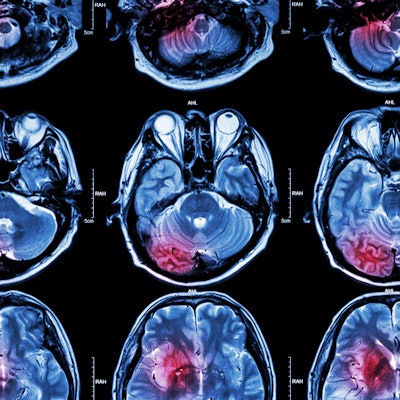
Researchers discussed how they used a deep-learning algorithm to detect, quantify, and assess the severity of infarcts in the brain on diffusion-weighted MRI (DWI-MRI) exams in acute ischemic stroke patients in a Sunday presentation at the virtual RSNA 2020 meeting.
A team of researchers led by presenter Seung Hyun Hwang of Yonsei University in Seoul, South Korea, developed a deep-learning model that can segment and quantify brain infarcts using DWI-MRI and then assess their severity by analyzing apparent diffusion coefficient (ADC) maps of the lesions. In testing, the model achieved high sensitivity and specificity.
"The qualitative and quantitative results of our study show feasibility for detecting and quantifying infarcts," Hwang said.
Due to its sensitivity for the detection of small and early infarcts, DWI-MRI is commonly used for evaluation of acute ischemic stroke, according to Hwang. ADC maps can also be used as a reference for acute infarction.
"However, it is dangerous to solely depend on ADC values, as ADC values are usually acquired at a single time point of the stroke in the course of clinical practice," he said.
Seeking to develop a deep learning-based automated infarct segmentation model, the researchers first gathered DWI and ADC maps from 394 patients with an acute infarct treated at their institution between January 2015 and May 2019. Of these datasets, 216 were used for training and 24 were utilized for validation. The remaining 154 datasets were set aside for testing of the model.
The team elected to utilize a modified U-Net convolutional neural network in an ensemble approach in order to improve performance on small lesions, according to Hwang. After segmenting the infarct, the algorithm then measures infarct severity based on analysis of the ADC maps of the lesions.
Infarcts are thereby classified into one of four categories: no stroke symptoms, minor stroke, moderate stroke, and severe stroke. An ADC value of more than 620 was used as the threshold for the "no stroke symptoms" category; other category thresholds were set at intervals of 100 ADC values.
In testing, the algorithm yielded:
- Average Dice coefficient: 0.85
- Average Dice coefficient when excluding extremely small lesions: 0.89
- Sensitivity: 83%
- Specificity: 99%
- Average volume difference for lesions calculated by model with those calculated by radiologists: 0.25 ml
"Our algorithm, which is an end-to-end segmentation model, can be easily deployed and applied for other segmentation tasks as well," Hwang said.
As their algorithm has so far only been tested on internal datasets, the researchers now plan to perform external validation of their model, Hwang added.
"Moreover, we plan to optimize our model in order to be more sensitive to small lesions," he said.