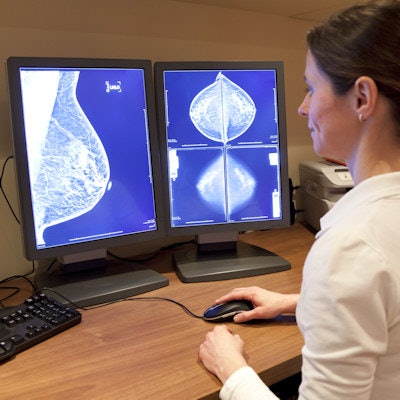
Artificial intelligence (AI) could effectively reduce the workload involved in reading mammography screening exams by eliminating studies most likely to be normal from the radiologist's worklist, according to a presentation delivered at ECR 2019 in Vienna.
The results suggest that it's feasible to use AI to cut radiologists' mammography reading workload, said presenter Dr. Kristina LĂĄng, PhD, of Lund University in Sweden.
"We wanted to see if we could identify normal mammograms in screening using artificial intelligence," LĂĄng said. "The rationale of this study was to find a simple and effective application of AI to improve mammography screening efficiency, and the aim was to evaluate if AI could reduce radiologists' workload of reading normal exams, and to assess its effect on false positives."
Deep learning
The study included data from a subset of the Malmö Breast Tomosynthesis Screening Trial, which consisted of 9,581 double-read mammography screening exams. Of these, 68 were screen-detected cancers and 187 were false positives, for a recall rate of 2.7% and a cancer detection rate of 7.1 per 1,000 exams.
The mammograms were analyzed with deep learning-based computer-aided detection (CAD) software (Transpara, ScreenPoint Medical), which categorizes them with a risk score of 1 to 10, with 10 being the highest risk of malignancy, LĂĄng said. The group then evaluated the performance of the AI algorithm in screening whether normal exams could be excluded, and what types of cancers the AI system missed. A team of three radiologists assessed the visibility of the cancers that AI missed.
Of the 9,581 exams, 5,082 (53%) had low-risk scores; of the 68 cancers identified, seven (10.2%) had low-risk scores; and of the 187 false positives, 52 (27.8%) had low-risk scores.
"The seven cancers with low-risk scores were all invasive, and all except one were clearly visible," LĂĄng said.
Characteristics of cancers missed or identified by AI | ||
Cancer type | Cancers scored as low risk missed by AI (n = 7) |
Cancers scored as high risk found by AI (n = 61) |
Invasive ductal carcinoma | 3 (43%) | 30 (49%) |
Invasive lobular carcinoma | 1 (14%) | 10 (16%) |
Invasive tubular carcinoma | 3 (43%) | 7 (11%) |
Ductal carcinoma in situ | 0 | 11 (18%) |
Other | 0 | 3 (5%) |
Overall, LĂĄng and colleagues found the following:
- 19% of screening exams were categorized as risk level 1 or 2. AI alone could safely read these.
- 69% of exams were categorized as risk level 3 through 9, and 31% of these were cancer. These could be single-read exams.
- 12% of screening exams were categorized as risk level 10, and 69% of these were cancer. These could be double-read exams.
Applying this type of protocol could not only reduce radiologists' workload but also screening costs and false positives, according to LĂĄng.
"An artificial intelligence system can reduce the screen-reading workload," she concluded. "With further improvement of the software, an even greater exclusion of normal mammograms seems possible since the majority of the cancers with low-risk scores were clearly visible."
Dealing with density
In other research presented in the same session, Alejandro Rodriguez-Ruiz of Radboud University Medical Center in Nijmegen, the Netherlands, and colleagues evaluated the relationship between the breast cancer detection performance of an AI system on mammography and breast density.
The study included a cancer-enriched database of 1,397 mammograms; of these, 179 were biopsy-verified cancers, and 1,218 were normal exams with at least two years of negative follow-up. The AI system scored each mammogram for the likelihood of cancer, and Rodriguez-Ruiz's team also calculated the breast density volume.
The study cohort was then divided into two groups based on density: high-density mammograms (73 cancers and 626 normal exams) and low-density mammograms (106 cancers and 592 normal exams). The team compared the area under the receiver operating characteristic curve (AUC) of the AI system between the two groups.
The researchers found that a mammogram's AI score did not correlate with density, and the AI system's AUC was similar for both groups (0.91 for the dense group compared with 0.89 for the nondense group). These results suggest that mammograms scored as normal can be considered as such regardless of density, Rodriguez-Ruiz and colleagues concluded.
"The AI score may be considered as an independent tool to estimate the likelihood of the presence of cancer on mammograms, to stratify screening populations, and to potentially speed the reading process by reassuring readers of mammograms that are likely normal, irrespective of breast density," the group wrote.