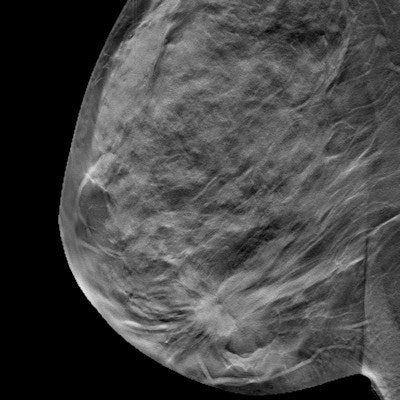
Digital breast tomosynthesis (DBT) remains one of the trickiest challenges for artificial intelligence (AI) algorithms. Could this challenge be surmounted if more DBT data were available in public databases? This question was tackled in an August 16 study in JAMA Network Open.
Part of the problem is that gathering a large volume of DBT data -- and making it public -- is a tricky task for many reasons, not the least of which are privacy requirements for medical information from patients, wrote a team led by Mateusz Buda of Duke University in Durham, NC.
"Medical imaging is a natural application of deep-learning algorithms," the group wrote. "However, well-curated data are scarce, which poses a challenge in training and validating deep-learning models."
Almost 40 million breast cancer screening exams are performed each year in the U.S. Although interest in using artificial intelligence with mammography is high, developing effective algorithms is difficult, particularly for 3D mammography.
To this end, Buda and colleagues sought to create an organized and annotated dataset of DBT images that could be used to create AI algorithms for breast cancer screening. (Annotation consisted of making note of biopsied masses or architectural distortions.)
The researchers gathered information from 16,802 DBT studies performed between August 2014 and January 2018 at Duke that included at least one reconstruction view. They then winnowed this data down to 22,032 reconstructed DBT images from 5,610 studies, making them anonymous and splitting them into training and test sets for a deep-learning model. The investigators evaluated the model's sensitivity with a threshold of two false positives per DBT volume.
These studies fell into the following four categories:
- 5,129 normal studies (91.4%)
- 280 studies which prompted additional imaging but no biopsy (5%)
- 112 biopsied studies that proved to be benign (2%)
- 89 studies with cancer (1.6%)
How did the model do? It showed a sensitivity of 65% at two false positives per DBT volume on a test set of 460 examinations from 418 patients -- a modest performance, but an improvement on previous research that reported sensitivity less than 20% at two false positives per volume, the group noted.
It's obvious that more work is necessary to make AI viable for use with 3D mammography.
"[This dataset is] a challenging but realistic benchmark for future development of methods for detecting masses and architectural distortions in DBT volumes," Buda and colleagues wrote.
The team has posted the dataset on the Cancer Imaging Archive, it said. It also plans to release its model to the public.
"[Making the dataset publicly available] will allow other groups to improve the training of their algorithms as well as test [them] on the same dataset, which could improve both the quality of the models and comparison between different algorithms," the authors concluded.
Important effort
The model's performance wasn't spectacular, wrote Dr. Joann Elmore of the University of California, Los Angeles and colleague Dr. Christoph Lee of the University of Washington in Seattle in an accompanying editorial. But using AI with 3D mammography is a difficult task.
"Compared with the reported performance of several commercial AI products for mammography, the performance of their model is not so great," the two noted. "However, tasking AI to detect breast cancer in DBT examinations, in comparison with two-dimensional digital mammograms, remains notoriously challenging."
Buda's group has taken on a valuable effort, Elmore and Lee wrote. To test and train an AI algorithm requires copious amounts of data and the development of AI algorithms has been blocked by a lack of large-scale, public datasets.
"Buda et al are bucking the trend and making their annotated image dataset publicly available, including their experiments' full code and network architecture with model weights," they noted. "The authors are to be commended for their scientific spirit and what we see as a sign of forward progress: scientists sharing data and code to advance the field of AI in medicine."