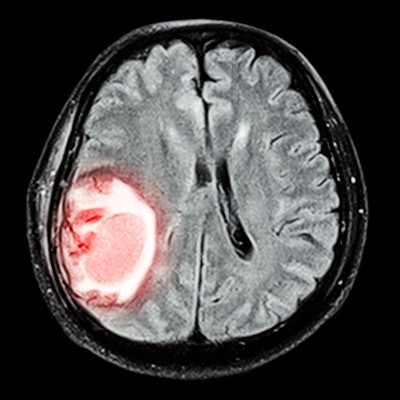
Artificial intelligence (AI) and radiomics can help to predict if a particular treatment strategy for acute ischemic stroke will be successful and how difficult the procedure will be, potentially paving the way for personalized endovascular patient management, according to research published online July 20 in Stroke.
A team of researchers led by Dr. Jeremy Hofmeister of Geneva University Hospitals in Switzerland developed two machine-learning algorithms that analyze radiomics features of clots shown on pretreatment noncontrast-enhanced CT scans. In prospective testing, the group's approach showed high levels of accuracy for predicting the first-pass success for a particular mechanical thrombectomy strategy and for estimating how many passes would be needed for successful recanalization.
"[We] have been able to show that quantitative information contained in the arterial occluding thrombus of patients with acute ischemic stroke can be useful in guiding the endovascular treatment of these patients," Hofmeister told AuntMinnie.com. "One of the [most interesting aspects] of this approach is that it is based on clinical images routinely performed to diagnose stroke, and therefore does not require any additional procedure for the patient... a crucial factor in emergency situations."
Mechanical thrombectomy has become the gold standard for treating acute ischemic stroke caused by large vessel occlusion. Although several endovascular treatment strategies are available for this procedure, there isn't currently a method for determining which technique would be best for a particular patient or to predict how difficult it will be to remove the clot.
As a result, the researchers sought to determine if radiomic features extracted from the clot on noncontrast-enhanced CT scans performed prior to intervention could be utilized to predict the likelihood of first-attempt recanalization and also to predict the overall number of passages needed to achieve successful recanalization with a mechanical thrombectomy device.
They first retrospectively gathered a cohort of 90 patients with acute ischemic stroke from their institution. After the thrombi were segmented on the noncontrast-enhanced CT studies, 1,485 thrombus-related radiomic features were then automatically extracted using the open-source 3D Slicer software. Nine radiomic features were found to be the most predictive of a successful recanalization.
Next, the researchers used these radiomic features to train two machine-learning models: one to predict first-attempt recanalization with thromboaspiration and the second to predict the overall number of passes needed with a mechanical thrombectomy device for a successful recanalization.
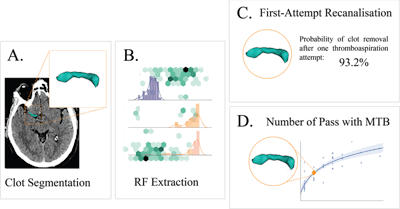
Performance of machine-learning models in acute ischemic stroke patients | ||
For predicting first-attempt recanalization with thromboaspiration | For predicting overall number of passes needed with a mechanical thrombectomy device for a successful recanalization | |
Results | Area under the curve = 0.88 | Explained variance = 0.70 Mean squared error = 0.76 Pearson correlation coefficient = 0.73, p < 0.05 |
"The algorithm is currently performing very well, and we believe it has the potential to provide a clinical gain in the speed of endovascular treatment of stroke patients," Hofmeister told AuntMinnie.com.
Once the thrombus has been segmented, the algorithm then processes the image data in just a few seconds, he said. However, the researchers acknowledged that their approach is currently limited by its reliance on manual segmentation of the thrombus, a process takes 15 to 20 minutes per patient.
Hofmeister noted, however, that several research teams are developing automatic thrombi segmentation software for acute ischemic stroke patients.
"Such systems would drastically reduce the total data processing time and allow the entire process -- segmentation and prediction -- to be performed very quickly and acceptably in an emergency clinical environment," Hofmeister said. "We hope to be able to implement this automatic segmentation quickly to test our method in clinical practice."