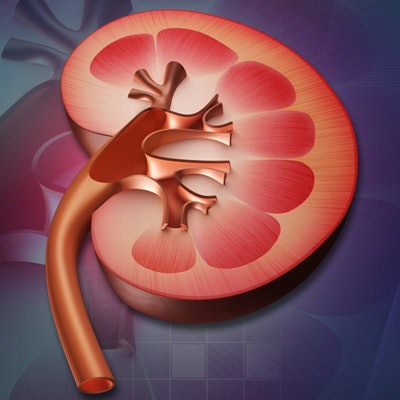
A machine-learning algorithm based on CT radiomics analysis can enable better preoperative risk classification of complex cystic renal lesions than relying only on the traditional Bosniak classification criteria, according to an international multicenter study presented Friday at the virtual RSNA 2020 meeting.
A research team from McGill University Health Center in Montreal and the Necker-Enfants Malades Hospital in Paris, France, developed a machine-learning algorithm that yielded 94% accuracy on an independent test for characterizing benign and malignant complex cystic renal lesions. In comparison, assessment based only on the Bosniak classification system for complex cystic renal lesions provided only 88% accuracy.
"Combining this radiomics approach with the Bosniak classification system into a decisional algorithm may help in avoiding unnecessary surgeries or follow-up," said presenter Dr. Jérémy Dana.
A common finding
Renal cysts are found in up to half of patients over 50 years old, and management and unnecessary long-term follow-ups or surgeries in these patients constitute a critical issue for healthcare systems, according to Dana. However, the commonly used Bosniak classification system continues to be limited by the suboptimal grading of lesion complexity using the IIF and III categories, he said.
The researchers hypothesized that quantitative image analysis of complex cystic renal lesions on contrast-enhanced CT images could accurately and reproducibly predict malignancy. They sought to retrospectively build and determine the diagnostic performance of a radiomics-based logistic regression model for the differentiation of benign from malignant complex renal cysts. Secondarily, they also hoped to propose a clinical decisional algorithm based on both the Bosniak classification system and the radiomics model to stratify patients for follow-ups and surgeries, Dana said.
First, they performed a database search at their institutions for patients who had cystic renal lesions larger than 1 cm between January 1998 and December 2018. The researchers sought to have a balance of Bosniak lesion categories in the datasets to ensure a harmonious distribution of complex cystic renal lesion semantic features, Dana said. Those with any history of intervention on the cyst or any condition associated with multiple renal cysts were excluded.
The reference standard was histological analysis or follow-up of more than four years by dedicated contrast-enhanced renal CT or MRI without any changes in the Bosniak classification. Radiological assessment of Bosniak classification was performed by an abdominal fellowship-trained reader with experience in urology imaging and a junior radiologist, blinded to pathology results and follow-up imaging.
Malignancy risk stratification
All renal cysts were segmented semiautomatically on contrast-enhanced CT images at the nephrogenic phase. Initially, 212 3D radiomic features were extracted from these segmented whole-volume renal cysts using the PyRadiomics Python package. After the 20 most important radiomics features for diagnosing cancer were determined, the researchers then trained and tested a random-forest classifier model to provide preoperative malignancy risk stratification.
The machine-learning algorithm was trained on a dataset of 149 patients from McGill University Health Center; testing was performed on an independent dataset of 50 patients from the Necker-Enfants Malades Hospital.
"Regarding the decisional algorithm, we intended to stratify Bosniak IIF and III lesions [ranging from] from 'certainly benign' with a distant follow up to 'likely malignant' with surgeries," Dana said.
Excellent diagnostic performance
They compared the results of the radiomics model with the diagnostic performance of the Bosniak classification system, which was calculated by regrouping patients into positive (categories III and IV) or negative (I, II, and IIF) results.
Performance on test set for characterizing complex cystic renal lesions on contrast-enhanced CT | ||
Bosniak classification system | Radiomics model | |
Area under the curve | n/a | 0.96 |
Sensitivity | 93% | 88% |
Specificity | 87% | 97% |
Accuracy | 88% | 94% |
Positive predictive value | 72% | 93% |
Negative predictive value | 97% | 94% |
"The machine-learning model achieved excellent diagnostic performance in distinguishing benign from malignant complex cystic renal lesions, outperforming the current Bosniak classification system," Dana said.
Delving further into the model's results, the researchers found the following:
- All Bosniak I and II cystic lesions were correctly classified as benign.
- The only malignant Bosniak IIF lesion was classified as benign, but with an elevated risk of 0.24.
- Three of the four Bosniak III lesions that were benign were correctly classified.
- Five of the six Bosniak III lesions that were malignant were correctly classified.
- All of the Bosniak IV lesions were correctly predicted with an average risk of 0.92.
Dana said that their model is robust, reproducible, interpretable, and simple.
"The proposed decisional algorithm, combining radiological readings of Bosniak categories with radiomics-based risk analysis, has shown potential in relieving the current burden of complex cystic renal lesions," he said.