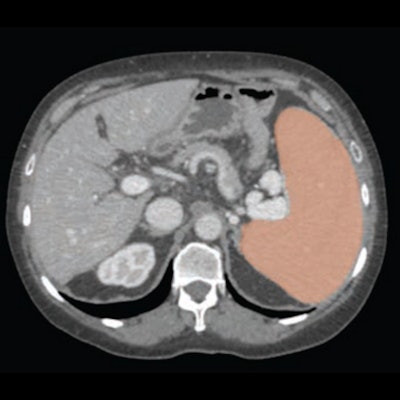
Using a deep-learning AI algorithm and weight-based volumetric thresholds with CT imaging shows promise in screening for enlarged spleen, a study published June 28 in the American Journal of Roentgenology has found.
The findings are good news for patients, especially those who have end-stage liver disease and are slated for a liver transplant, wrote a team led by Alberto Perez, MD, of the University of Wisconsin School of Medicine in Madison.
"The AI tool could facilitate opportunistic screening for splenomegaly," the group wrote.
Enlarged spleen can be caused by infectious, hematologic, neoplastic, congestive, or inflammatory conditions, and may be an "early finding of an underlying disease process ... [making] accurate diagnosis of splenomegaly ... critical," the investigators noted.
Estimating splenic volume has traditionally been done via linear measurements, but this approach has its limitations, according to Perez and colleagues. To explore assessing the spleen with volumetric analysis, the group conducted a study that included a screening cohort of 8,901 patients who underwent CT colonoscopy or renal-donor CT between April 2004 and January 2017. The team used a deep-learning algorithm that was developed, trained, and tested at the National Institutes of Health (NIH) Clinical Center to perform spleen segmentation and determine organ volumes; two radiologists reviewed these segmentations.
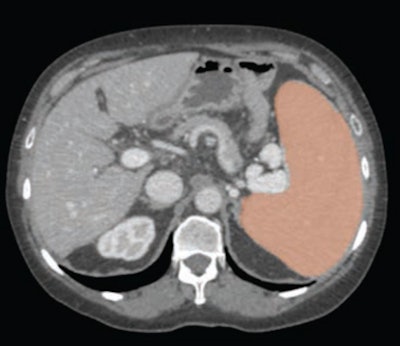
The team reported that the AI algorithm effectively calculated splenic volumes from CT exams in 8,853 patients from the primary cohort of 8,901 (99%), and that splenic volume had a statistically significant association with patient weight.
The investigators also found the following:
- Both radiologist readers confirmed enlarged spleen in 20 patients who had a splenic volume of zero as determined by the deep-learning algorithm.
- Mean automated splenic volume was 216 ml.
- Sensitivity and specificity for volume-defined splenomegaly were 13% and 100% at true-craniocaudal length of 13 cm, and 78% and 88% for maximum-3D length of 13 cm.
The effective performance of the deep-learning algorithm for evaluating splenic volume could be an important additional tool for patient assessment, according to the researchers.
"Use of the automated deep-learning AI tool and weight-based volumetric thresholds could
allow large-scale evaluation for splenomegaly on CT examinations performed for any indication," they concluded.
The complete study can be found here.