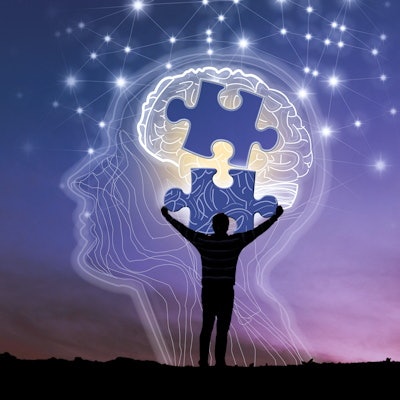
Adding MRI features to clinical ones in a machine learning model can help predict the progression of Alzheimer's disease among those with early disease, according to a study published on December 13 in Alzheimer's & Dementia.
"Our validated prediction models enable baseline prediction of clinical progression trajectories in early AD, benefiting clinical trial enrichment and various applications," wrote a team led by Viswanath Devanarayan, PhD, of Eisai in Nutley, NJ.
Models for predicting Alzheimer's disease progression are needed to both make clinical studies more effective and to monitor patient treatment. But this task can be challenging due to differences in patients' baseline cognitive function, which includes clinical factors such as word recall and executive skill as well as brain MRI measures or biomarkers, the authors explained.
"If such baseline patient characteristics can be used collectively to build prognostic prediction models to predict the future clinical decline of each patient, such predictions during initial patient screening can be used to design optimal clinical trials and as a guide for treatment, monitoring, and patient care decisions in clinical practice and real-world situations," they wrote.
The researchers investigated whether AI could help forecast Alzheimer's progression, creating machine learning prediction models using a training cohort (934 patients) and two validation cohorts (group 1, 235 patients and group 2, 421 patients). The models included features such as cognitive function assessments, APOE ε4 status, and patient demographics as well as brain MRI data. (All participants in the training cohort and group 1 validation cohort underwent 3-tesla MRI, while 75% of those in the second validation cohort underwent 1.5-tesla MR imaging and the rest received 3-tesla imaging. These exams assessed brain volume, area, and cortical thickness.)
Adding MRI features to clinical ones improved the performance of the models, measured by the square of the regression line, or R2 (with 1 as reference).
Comparison of models to predict two-year cognitive decline in early-stage Alzheimer's patients
Square of the regression line (R2) | Clinical features alone | Clinical and MRI features combined |
Validation cohort 1 | 0.21 | 0.29 |
Validation cohort 2 | 0.31 | -- |
"Adding MRI features to this model resulted in a notable improvement in [validation group 1, which employed the same image processing pipeline as [the training cohort]," the investigators reported. "However, this enhancement in model performance was not replicated in [validation group 2], where differences in the image preprocessing methods between [the training cohort] and [validation group 2] likely had an impact."
The results show promise for predicting Alzheimer's disease progression, although more research is needed, according to the authors.
"These models can readily accommodate other types of baseline assessments such as those from MRI, PET, and fluid biomarkers to improve prediction performance," they concluded. "These prognostic predictions have a wide-ranging impact across clinical drug development, clinical practice, and real-world applications."
The complete study can be found here.