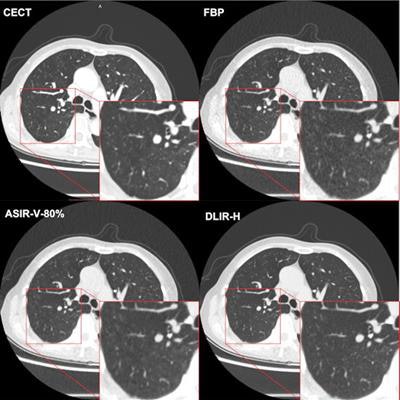
A deep learning-based image reconstruction algorithm can enable detection of more lung nodules on ultralow-dose CT exams -- at radiation doses comparable to a chest x-ray, according to a study published January 18 in Radiology.
A team of researchers led by Beibei Jiang of Shanghai Jiao Tong University School of Medicine prospectively assessed three different image reconstruction techniques for their image quality and ability to detect lung nodules on ultralow-dose CT exams. The group found that deep learning-based reconstruction outperformed filtered back projection (FBP) and adaptive statistical iterative reconstruction (ASIR-V) for both metrics.
"Compared with adaptive statistical iterative reconstruction-V, deep learning image reconstruction ... improved the image quality by reducing image noise and increasing the lung nodule detection rate," the authors wrote. "[Deep learning image reconstruction] also improved the nodule measurement accuracy and displayed more detailed malignancy-related imaging features of nodules."
Although ultralow-dose CT could facilitate the implementation of large-scale lung cancer screening while minimizing the radiation dose for patients, traditional image reconstruction methods have been associated with image noise in low-dose image acquisitions, according to the researchers.
To determine if deep learning-based image reconstruction could help, the authors prospectively studied 203 consecutive patients at Shanghai General Hospital from April to June 2020 who had received noncontrast ultralow-dose CT performed at 0.07 or 0.14 millisieverts -- similar to the level of a single chest radiograph -- as well as a contrast-enhanced CT exam. All ultralow-dose CT images were reconstructed with filtered back projection, ASIR-V, and a commercial deep learning-based image reconstruction algorithm (TruFidelity, GE Healthcare).
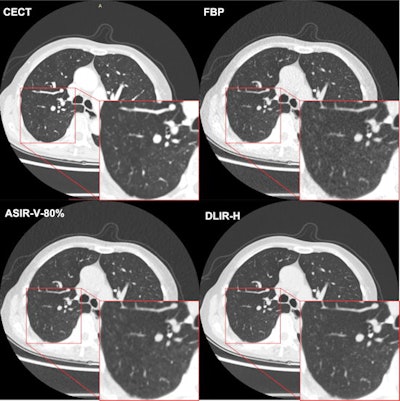
Next, the researchers performed 3D segmentation of the lung tissue to assess image quality. A radiologist then used deep learning-based nodule evaluation software (InferRead CT Lung 8.6, Infervision) to detect and measure the long diameters and volumes of the lung nodules on all four types of images. These findings were later independently confirmed by another radiologist with 20 years of experience.
Impact of deep-learning image reconstruction on lung nodule detection | ||
ASIR-V | Deep-learning image reconstruction | |
Background image noise | 29 Hounsfeld units | 23 Hounsfeld units |
Nodule detection rate | 781 of 1,066 (73%) | 808 of 1066 (76%) |
Percentage difference of long diameter compared to contrast-enhanced CT | 9.2% | 6.2% |
"With the introduction of this specific [deep learning image-reconstruction] algorithm, it is feasible to use chest radiography-equivalent chest CT acquisition as low as 0.14 mSv in underweight and normal-weight participants to detect and characterize lung nodules, which is crucial to facilitate the clinical implementation of large-scale lung cancer screening," the authors wrote.
They noted, however, that only a limited number of nodules had pathologic results; a larger sample size may have yielded different results.
"More studies are needed to explore the clinical application and image optimization of [deep learning image reconstruction]," the researchers wrote.
In an accompanying editorial, Dr. Ho Yun Lee of the Sungkyunkwan University School of Medicine in Seoul, South Korea said that the study "adds to the growing body of clinical research now positioning the use of [deep-learning image reconstruction] in a variety of lung disease situations -- especially those that require repeated use of [ultralow-dose] CT, like COVID-19."