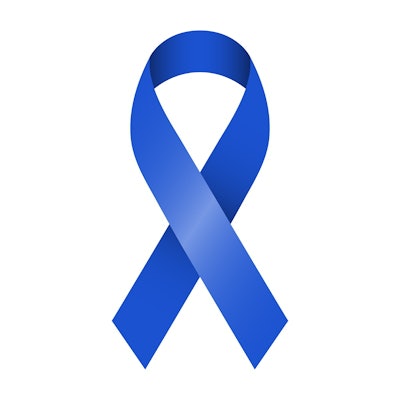
Radiomics analysis of pretreatment CT exams can help to predict response to neoadjuvant chemoradiation therapy in patients with locally advanced rectal cancer, according to research presented at last week's ECR 2022 Overture.
Researchers from China led by Dr. Xiaoping Yi of Central South University in Changsha developed a predictive model that encompasses analysis of radiomics, clinical, and pathological variables. In testing, the model yielded an area under the curve (AUC) of 0.872 for predicting treatment response from pretreatment CT studies.
"Our data support using [routine] noninvasive imaging-focused approaches to assess treatment response and to guide personalized treatment for patients with rectal cancer," Yi said.
In locally advanced rectal cancer, the standard treatment is neoadjuvant chemoradiotherapy, followed by total mesorectal resection. However, response to treatment can vary widely, ranging from pathological complete response to virtually no tumor regression or even progression, according to Yi.
As conventional analysis of MRI and CT exams remains limited for predicting treatment response, there is currently an unmet need for noninvasive imaging methods that can provide this capability, according to Yi.
"Both CT and MR radiomics show potential in predicting treatment response in locally advanced rectal cancer, but the results were still controversial and far from conclusive," he said. "More research is needed to evaluate CT radiomics as a strategy for predicting treatment response."
In their study, the researchers sought to assess the benefits of radiomics analysis of pretreatment CT images for predicting pathological complete response. They used the least absolute shrinkage and selection operator (LASSO) method for identifying radiomics features and then trained a predictive model that analyzes radiomic, clinical, and pathological variables.
They gathered data from 216 consecutive patients at their institution with locally advanced rectal cancer. Of these 216, 44 (20.4%) achieved pathological complete response.
The researchers utilized 151 of the cases for training of the predictive model and 65 for validation. Both patient cohorts were similar in terms of pathological complete response rate and other clinical and pathological variables, according to Yi.
Specifically, the predictive model analysis included the CT radiomic signature, the distance to anal verge on CT; the lymphocyte-to-monocyte ratio; and carcinoembryonic antigen results. The model yielded an AUC of 0.926 on the training cohort and 0.872 on the validation cohort.
"Our predictive model, built with a combination of pretreatment radiotherapy planning CT-based radiomics and clinicopathological risk factors, had reasonable performance in differentiating patients with and without [pathological complete response]," Yi said. "The clinical usefulness of this model was also confirmed."
In comparison to other studies in the literature reporting on the use of CT radiomics in this application, the group's model is more interpretable and benefitted from larger sample sizes for training, he said.
"Our results support further investigation of the [radiomics features] as a biomarker to predict the response of [locally advanced rectal cancer] to neoadjuvant chemoradiotherapy," he said.
Yi also noted that the clinical and pathological characteristics of the model were also essential for the predictive model's classification efficiency.