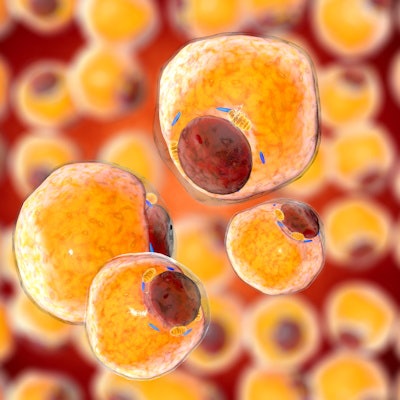
With help of artificial intelligence (AI), CT-based body composition analysis shows utility for a variety of important clinical applications, according to a presentation at the annual American Roentgen Ray Society (ARRS) meeting in New Orleans.
For example, AI-derived body composition measures can be utilized to spot cardiovascular disease, predict the risk of developing certain diseases and outcomes, and help in providing appropriate dosing levels in chemotherapy, said Dr. Kirti Magudia, PhD, of Duke University.
"Robust large-scale automation of CT-based body composition analysis is feasible," she said in her talk on the potential for body composition analysis in abdominal imaging applications.
CT-based body composition analysis is a form of opportunistic screening that has relevance to cardiovascular disease and cancer, according to Magudia. In contrast to organized screening coordinated by the public sector, opportunistic screening depends on requests from patients or their providers and takes advantage of when a patient presents for care (and imaging) for another indication.
There are two main categories currently being explored for utilizing AI in opportunistic screening on CT: the development of clinically significant biomarkers (such as for coronary calcium scoring) and identification of incidental findings (such as automated detection of abdominal aortic aneurysms), according to Magudia.
Traditionally, body mass index (BMI) has been used to provide insight into body composition and has been associated with survival and cardiovascular risk. However, there is variability on a per-patient basis; two patients with identical BMI could have different ratios of subcutaneous fat to skeletal muscle, for example, she said.
Among body composition measures, subcutaneous fat has been associated with eccentric left ventricular remodeling as well as chemotherapy toxicity, for example. In addition, subcutaneous fat and visceral fat have been tied to insulin resistance and increased risk of various malignancies.
Furthermore, visceral fat and skeletal muscle area have been associated with eccentric left ventricular remodeling, longer cardiac output, and higher systemic vascular resistance. And skeletal muscle area has also been tied to survival in patients with lung, gastrointestinal, metastatic renal, and metastatic breast cancer, she said. Magudia noted that body composition varies by age, sex, and gender.
Traditionally, body composition has been measured by calculating areas of subcutaneous fat, visceral fat, and skeletal fat areas on a single axial CT slice at the L3 vertebral level. Manual analysis is the standard approach, but is time-intensive and costly, according to Magudia. Consequently, its use has been largely limited to small cohorts and well-funded research studies, she said.
AI can help, however, and many groups in the U.S. and around the world are working on developing AI methods for body composition analysis. At Duke, researchers have developed automated body composition analysis software that identifies the axial CT series, segments a single slice at the level of the L3 vertebral bodies, and then provides a segmentation map with body composition measures.
They also have defined normal values for body composition, specific to age, race, and sex. These normalized body composition measurements were found to be clinically significant in a recent study.
Patients in the highest quartile of visceral fat area had the highest risk for myocardial infarction. Furthermore, patients in the lowest quartile for visceral fat area had the lowest risk for stroke. In other findings, patients in the lowest quartile of skeletal muscle area had lower two-year survival rates.
In the future, these CT-based normalized body composition measures need to be validated in other race/ethnicity groups and other geographic areas, she said.
"My ultimate goal is to hopefully have this data available for all acquired abdominal CT scans and electronic medical records," Magudia said.