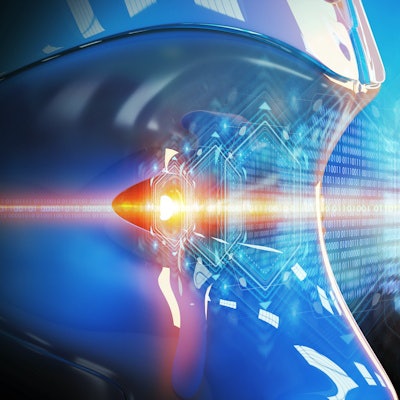
Clinical use of radiomics and artificial intelligence (AI) in genitourinary (GU) applications has been limited to date, but that's likely to change in the future, according to a talk at the American Roentgen Ray Society (ARRS) meeting in New Orleans.
Radiomics and AI will increasingly be part of genitourinary imaging for both research and clinical practice, according to Dr. Satheesh Krishna of the University of Toronto.
"These tools will initially augment, but may eventually replace, certain radiologists' tasks, which can improve efficiency and diagnostic accuracy," said Krishna, who presented the talk on behalf of Dr. Nicola Schieda of the University of Ottawa.
Hundreds of research studies have reported on the use of radiomics in prostate cancer, ranging from tasks such as tumor detection, grading, and staging, as well as predicting biochemical recurrence of cancer. The data, however, consist mostly of small to moderately sized, single-center retrospective studies that lack broad reproducibility, according to Krishna.
Researchers have also developed machine-learning algorithms to provide whole-gland and zonal anatomy segmentation of the prostate, as well as tumor detection and segmentation, he said.
In other GU applications, the kidney has also been a popular research area for radiomics, Krishna said. These efforts include identification of solid and cystic renal masses.
"Not unlike the prostate, these are also mainly single-center retrospective studies in highly selected cohorts without external validation," he said. "These are big limitations on the literature."
Machine learning is also emerging for use in the kidney to perform tasks such as renal segmentation, as well as renal cyst and tumor detection and classification, according to Krishna.
Other radiomics and machine-learning tools are being investigated for a wide variety of other genitourinary applications.
"It should not be surprising that radiomics and machine learning has and is being used extensively in every structure of the genitourinary tract from the adrenals to the reproductive organs," he said. "Just keep in mind that the more obscure the organ or the disease, the smaller the case numbers and the more limited radiomic and machine-learning applications will be."
What types of these tools are currently available for use in clinical practice? Not many, Krishna said.
As of now, the only machine learning-based software for GU applications to have received regulatory approval are designed for use in the prostate. These software tools, which perform automated segmentation and/or tumor detection on MRI, include the following:
- Prostate MR (Siemens Healthineers): Prostate gland segmentation and lesion detection -- U.S. and Europe
- ProView (GE Healthcare): Prostate gland segmentation and lesion detection -- U.S. and Europe
- Quantib Prostate (Quantib): Prostate gland segmentation and lesion detection -- U.S. and Europe
- OnQ Prostate (Cortechs.ai): Prostate gland segmentation -- U.S.
- QP-Prostate (Quibim): Prostate gland segmentation -- U.S.
- JPC-01K (JLK): Prostate lesion detection -- Europe
As for other organs, radiomics and AI tools for other organ systems remain primarily investigational at this time and are mostly available on open-source platforms such as GitHub, Krishna said.
Krishna noted that future research in this area should strive to standardize assessment, processing, output, and results with multicenter external validation and large sample sizes.
Also, "clinical [products require] robust validation before and after regulatory body approval," he said.