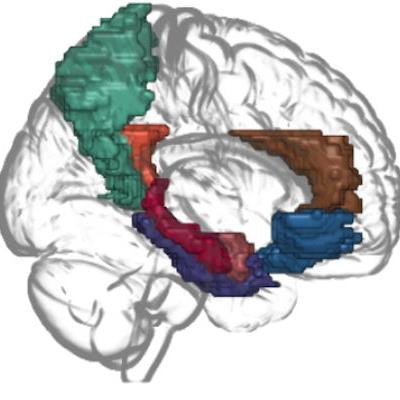
The combination of radiomics features on functional brain MRI (fMRI) exams and a machine-learning algorithm can identify social anxiety in young adults with a promising level of performance, according to research published August 17 in Scientific Reports.
In a study involving over 100 young adults, a team of researchers led by Byung-Hoon Kim of Yonsei University College of Medicine in Seoul, South Korea, found that a machine-learning model achieved nearly 80% accuracy for classifying participants as either having low or high social anxiety based on analysis of resting-state brain functional radiomics features.
"Our approach based on machine learning with the functional radiomic features for predicting the level of social anxiety may potentially be a useful screening tool for people with high level of social anxiety, and possibly other psychopathologic phenotypes in the future," the authors wrote.
Using an ad on the internet, the researchers recruited 116 young adults who were not currently receiving psychiatric treatment or had any history of diagnosis or treatment with a major psychiatric disorder. On psychiatric testing, the participants had a mean Liebowitz Social Anxiety Score (LSAS) score of 54.3 with a standard deviation of 28.3, indicating a wide variety of social anxiety levels, according to the researchers.
All patients received T1-weighted and fMRI scans at one of two institutions on either an Ingenia CX (Philips) 3-tesla scanner or a Magnetom Verio 3-tesla scanner (Siemens Healthineers). Radiomics features were extracted using the open-source Analysis of Functional NeuroImaging (AFNI) software.
Next, they trained six machine-learning models (logistic regression, support-vector machine, random forest, multilayer perceptron, and extreme gradient boosting [XGBoost]). These algorithms were trained to analyze six radiomics features: regional homogeneity, fractional amplitude of low-frequency fluctuation, fractional resting-state physiological fluctuation, and degree centrality of the resting-state fMRI within the orbitofrontal cortex, anterior cingulate cortex, posterior cingulate cortex, hippocampus, parahippocampal gyrus, and amygdala.
The best-performing machine-learning algorithm -- XGBoost -- achieved a balanced accuracy of 77.7% and an F1 score -- a measure that reflects sensitivity and specificity by computing the harmonic mean of precision and recall scores -- of 0.815.
"Although the accuracy for prediction may be a little below the certain point that the method can actually be extended to clinical practice, the experimental results still hold the significance in that they validate the potential possibility of radiomics-based machine learning for screening young adults with high social anxiety who were not aware of, or not able to seek clinical help," the authors wrote.
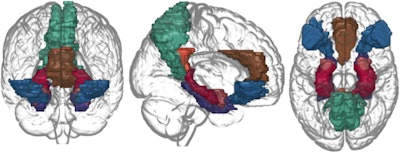
After analyzing the importance of each of the radiomics features, the researchers concluded that the brain regions responsible for high-level functions were more important to the social anxiety predictions than those related to low-level limbic functions. The degree centrality was the most important radiomics feature for the machine-learning classifier, while orbitofrontal cortex, amygdala, and the parahippocampal gyrus were the three most important regions for classifying social anxiety.
The authors noted that these findings demonstrate that the model is appropriately analyzing the activity level and connectivity of the orbitofrontal cortex and amygdala -- factors known to be related to the level of social anxiety in patients with social anxiety disorder.