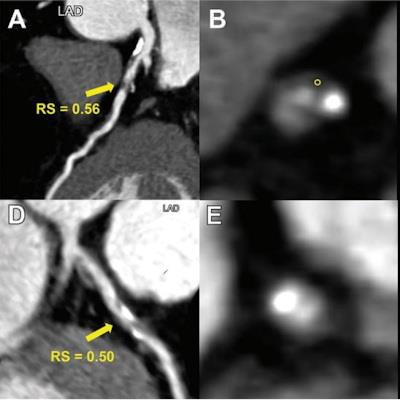
Researchers have developed a radiomics model using coronary CT angiography (CCTA) features that can identify plaques linked to increased risk for future adverse cardiac outcomes.
A group led by Dr. Qian Chen from Nanjing Medical University in China reported that the model had "moderate to good" diagnostic performance, and in an independent cohort study, found that the radiomic signature was linked to increased risk for major cardiac events. Their results were published February 14 in Radiology.
"The proposed radiomic signature offers incremental predictive value of adverse outcomes and may serve as a promising noninvasive imaging marker in risk stratification in future clinical practice," Chen and colleagues noted.
In most cases of acute coronary syndrome, large, lipid-rich plaques are ruptured, identifying these plaques important for treatment. Intravascular ultrasound can visualize plaque features, but the researchers pointed out that this method is invasive and not widely available.
CCTA is a first-line imaging method for diagnosing coronary artery disease. But the researchers explained that conventional parameters don't assess heterogeneity and spatial complexity in plaques. Radiomics, meanwhile, takes "thousands" of features from medial images and factors them into automated, data-driven evaluation. Several small previous studies report the potential that radiomics based on CCTA has in cardiac imaging.
Chen and colleagues wanted to explore whether a radiomic signature based on CCTA features could detect vulnerable plaques, as well as see whether these plaques are tied to risk for future major adverse cardiac events. They used intravascular ultrasound as the reference standard for their model.
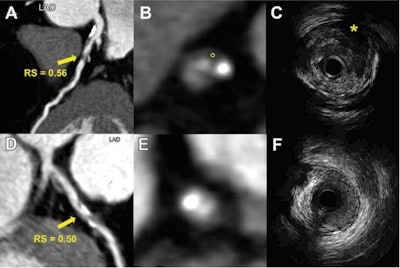
The radiomic signature development data included patients first undergoing CCTA and then intravascular ultrasound within three months between 2013 and 2020 at one hospital. In total, the researchers included 419 lesions from 225 patients with an average age of 64. The model used 16 radiomic features, including two shape features and 14 textural features for its development.
The team also evaluated the model's prognostic value for predicting major adverse cardiac events in a prospective cohort, using data collected between 2018 and 2019 from 1,020 lesions from 708 patients with an average age of 62.
Chen and colleagues reported that the model achieved the following area under the curve (AUC) values: training set, 0.81; validation set, 0.75; internal test set, 0.80; and external test set, 0.77. The investigators also found that a high radiomic signature of greater than or equal to 1.07 was independently tied to major adverse cardiac events over a median three-year follow-up period (hazard ratio, 2.01; p = 0.005).
In a news release, the investigators wrote that they intend to build a radiomics model from different scanner types and vendors, and they are planning a larger, multicenter study of 10,000 patients, which they said would help guide clinical decision-making and improve patient care in the future.
In an accompanying editorial, Dr. Carlo De Cecco, PhD, and Marly van Assen, PhD, from Emory University wrote that this work is "an important step forward" in realizing patient-centered models for cardiac disease. They added that it opens opportunities to larger clinical trials validating these radiomic signatures in different settings and populations.
"This study shows compelling data to encourage further investigation of the use of radiomic features to identify high-risk plaque profiles," de Cecco and van Assen wrote. "Incorporating data from the electronic medical records to imaging features and radiomics data can further personalize cardiovascular care."