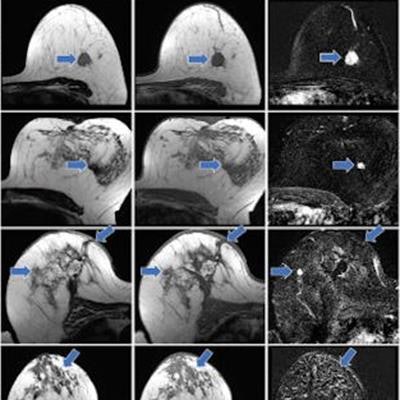
Machine learning could make way for reducing the contrast dose needed for breast MRI, according to a study published March 21 in Radiology.
A team led by Gustav Müller-Franzes from University Hospital RWTH Aachen, found that generative adversarial networks (GANs) can use simulated low-contrast-enhanced images to recover the full image in contrast-enhanced breast MRI.
"When the generated and real images were compared side by side, lesion conspicuity on synthetic images was noninferior," the Müller-Franzes team wrote. "When GANs used only the pre-contrast images as inputs, experienced radiologists were more often able to distinguish synthetic from real images, and lesion conspicuity was inferior."
While controversial, gadolinium-based contrast agents are used in some breast MRI exams, especially for women with extremely dense breasts. The researchers noted clinicians' interest in reducing the amount of contrast needed for breast MRI and wrote that machine learning is one method being explored for this purpose.
Previous research has suggested that machine learning may allow for a reduction in gadolinium dose in brain MRI exams without compromising image quality. Müller-Franzes and colleagues wanted to determine if GANs, which can generate images using machine learning, could recover contrast-enhanced breast MRI scans from unenhanced images and virtual low-contrast-enhanced images.
They tested this via two approaches in a study that included 9,751 breast MRI exams from 5,086 women. The first approach (A) used GANs trained to recover contrast-enhanced images from simulated low-contrast images. The other (B) had GANs recover contrast-enhanced images from unenhanced T1- and T2-weighted images. Two experienced radiologists used both approaches to try to distinguish between real and synthesized contrast-enhanced images with two independent sets of 100 images each.
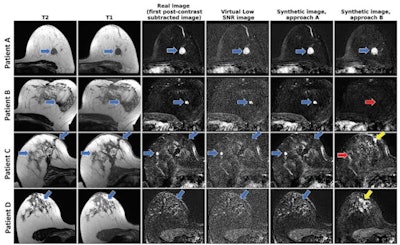
Müller-Franzes and colleagues found that the radiologists could not distinguish the synthetic images from the real post-contrast-enhanced images. Radiologist 1 was correct in choosing 49 of 100 images while radiologist 2 chose 55 out of 100 images correctly. The study also showed that, when using images generated according to approach B, the two radiologist readers correctly determined whether an image was real or synthetic for 67 of 100 images (p < 0.001) and 55 of 100 (p = 0.18).
When the readers directly compared the synthetic images from approach A with the real images side by side, their average ratings on a Likert scale were 4.5 and 4.7 -- confirming the finding that the synthetic images were noninferior to the real ones.
When performing the same experiment with the synthetic images generated by approach B, the two radiologists reported average Likert scale ratings of 3.3 and 2.8, a result that did not meet the predefined noninferiority criterion, the investigators noted.
Finally, the study showed that on a Likert scale, the average ratings of lesion conspicuity on synthetic images from approach A were significantly higher than those generated according to approach B (4.9 vs. 1.8, p < 0.001).
Müller-Franzes and colleagues called for future studies to explore the practical feasibility of using GANs in this setting.