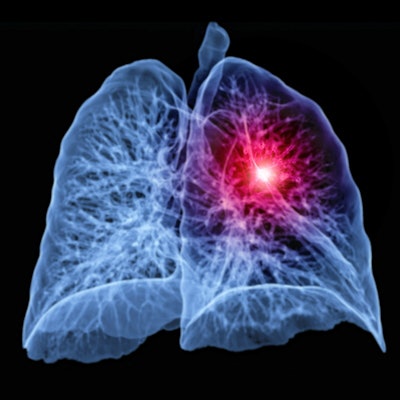
Body composition measurements from preoperative CT images can help determine if patients with lung cancer will experience recurrence after surgery, according to research published April 7 in Lung Cancer.
A team of researchers led by Dr. Naciye Gezer from the University of Pittsburgh retrospectively studied 363 lung cancer patients using a model comprising CT radiomics analysis, clinical features, and pathology findings. In testing, they found that the model yielded a promising level of accuracy for predicting three-year recurrence.
"This study demonstrated that the prediction of lung cancer recurrence after curative intent surgery could be improved by using radiomic, clinical, and pathologic factors," the authors wrote.
In patients with early and locally advanced non-small cell lung cancer (NSCLC), surgical resection is considered to be the optimal treatment. However, up to over half of patients receiving resection experience cancer recurrence and poor outcomes.
"Identification of additional factors that influence postoperative cancer recurrence has the potential to allow better prediction of the likelihood of recurrence and thereby personalize both surveillance strategy and therapy," the authors wrote.
To test their hypothesis that body composition may be related to cancer recurrence, the researchers assembled a cohort of 363 lung cancer patients who had underwent lung resections and were followed up for at least six years. Of the 363 patients, 242 had recurrence after surgery, and 173 had cancer-related death. An additional 104 patients died from other causes during the median follow-up time of 7.5 years.
Using an internally developed convolutional neural network, five body tissues were automatically segmented on a preoperative whole-body CT scan acquired during a PET/CT exam. Another algorithm was used to automatically segment ten tumor features on preoperative dedicated chest CT studies. Their analysis also included clinical, demographic, and histopathological information.
In addition to compiling hazard ratios (HRs) of normalized individual variables to evaluate their significance alone and in combined models, the researchers performed fivefold cross-validated time-dependent receiver operating characteristic analysis to assess the ability to predict lung cancer recurrence, focusing mostly on a three-year time window.
The researchers found that five body tissues showed standalone potential for predicting lung cancer:
- Visceral adipose tissue volume: Hazard ratio (HR) = 0.88 (p = 0.047)
- Subcutaneous adipose tissue density: HR = 1.14 (p = 0.034)
- Inter-muscle adipose tissue volume: HR = 0.83 (p = 0.002)
- Skeletal muscle density: HR = 1.27 (p < 0.001)
- Total fat volume: HR = 0.89 (p = 0.05)
Furthermore, CT-derived tumor features such as ground-glass opacity, calcification, and cavity ratio parameters were also associated with postoperative cancer recurrence.
A model that combined analysis of clinicopathologic data and radiomics features delivered the best overall performance, yielding an area under the curve (AUC) of 0.78 for predicting recurrence within three years.
"Our investigation showed that lung cancer recurrence was associated with both CT-derived body composition and tumor-specific characteristics," the authors concluded. "These features also improved the prediction of recurrence compared to standard clinicopathological factors, especially for the identification of subjects at low risk of recurrence, at least in the first three years after surgery. The unacceptably high rate of lung cancer recurrence after surgery makes this study critically important to reveal the underlying mechanisms behind these factors, which may ultimately facilitate individualized surveillance and therapy."