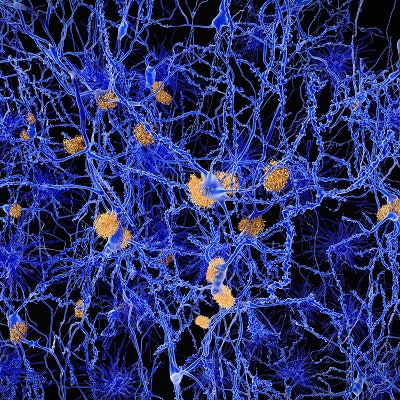
Machine-learning technology that considers clinical, imaging, proteomic, and genomic parameters shows potential for the early diagnosis of Alzheimer's disease -- before symptoms begin to affect daily living, according to research published online August 15 in Scientific Reports.
A team from Case Western Reserve University has developed a machine-learning algorithm that integrates a range of Alzheimer's disease indicators -- including mild cognitive impairment -- and determines which variables are best for the classification task. In testing of its ability to detect Alzheimer's disease, the algorithm outperformed individual indicators alone as well as methods that simply combined them all together without any selective assessment.
What's more, the Case Western algorithm was also better at predicting mild cognitive impairment than other methods, according to the group.
The algorithm, called cascaded multiview canonical correlation (CaMCCo), assesses measurements from MRI, features of the hippocampus, glucose metabolism rates in the brain, proteomics, genomics, mild cognitive impairment, and other parameters. In a two-stage process, the algorithm selects the most pertinent parameters to predict who has Alzheimer's, according to the team.
"The algorithm assumes each parameter provides a different view of the disease, as if each were a different set of colored spectacles," senior author Anant Madabhushi, PhD, said in a statement from the university.
It first selects the parameters that best distinguish between healthy and unhealthy subjects. Next, the algorithm selects from the unhealthy variables those that best distinguish who has mild cognitive impairment and who has Alzheimer's disease.
"The remaining views are combined to give the best picture," Madabhushi said.
The Case Western team, which included Asha Singanamalli and Haibo Wang, PhD, tested CaMCCo using data from 149 patients collected via the Alzheimer's Disease Neuroimaging Initiative (ADNI). The algorithm's approach of fusing select modalities for each classification task produced a mean area under the curve (AUC) of 0.92, outperforming fusion of all modalities (mean AUC = 0.54) and the individual modalities alone (mean AUC = 0.90, 0.73, 0.71, 0.68, 0.62, and 0.53). In addition, the algorithm yielded an 80% positive predictive value for predicting mild cognitive impairment, besting two other multiclass classification methods that offered only 67% and 63% positive predictive values.
The researchers are continuing to validate and fine-tune the algorithm using data from multiple sites. In addition, they plan to use the software in an observational capacity; the computer would run the data as a collaborating neurologist compiles tests on patients. If the algorithm proves successful for the early prediction of Alzheimer's disease, Madabhushi said he expects to pursue a clinical trial for prospective validation.