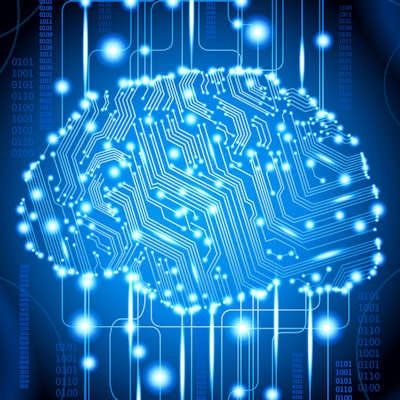
Artificial intelligence (AI) can enhance the business and operational side of radiology, but radiologists and practice managers should understand the technology before they consider adopting it, according to a talk on April 5 at the Radiology Business Management Association's (RBMA) Paradigm annual conference in San Diego.
By being educated on the benefits and limitations of the underlying AI technology and the kinds of problems that can be successfully addressed with machine learning, radiology professionals will be able to properly evaluate what the technology can do for them, said Dr. R. Kent Hutson, a neuroradiologist at Radiology and Imaging Consultants in Colorado Springs, CO.
"You have to be able to ask the right questions and understand how the data are being used to take advantage of the tools," Hutson said.
Nonimage interpretation tasks
The use of AI for image interpretation tasks has generated most of the hype in radiology, but the technology will have the most impact on other applications in the short term, according to Hutson. For example, machine-learning algorithms could be deployed in billing and collections, ensuring that more-accurate claims could be submitted for reimbursement -- reducing the number of denied claims.
"If you look at credit card companies and large financial institutions, they've been using these same kinds of techniques for a very long time," he said. "That's where a lot of these techniques really started being used commercially, in analyzing the customer, determining who's going to be the best customer, [and] who are the customers at risk for leaving the company."
Machine learning could also be used to enhance the operation of a radiology department, such as by optimizing scheduling and helping to make radiologists as efficient as possible by addressing inefficiencies in their daily workflow, Hutson said. This includes optimizing hanging protocols to help radiologists get through studies faster.
"Any time spent having to rearrange things or doing anything at the workstation other than looking at pictures and dictating the report is going to translate to lost time and lost revenue," he said. "Any computer-based way of making that more efficient is a benefit. You don't have to use machine learning to do all of these things, but machine learning is really a different framework for programming that tends to make more complex processes easier to program, easier to solve with a computer."
Does it work?
It's vital, though, that prospective adopters of AI understand what problems it can actually solve. The first step is determining if the question the AI technology is being asked to answer is well-defined and if the question can be answered by a computer. Next, users need to understand the data requirements for an algorithm to be used in a given application, Hutson said.
"Intuitively, can we answer this question with the data we have, and do we have the right types of information about this problem to be able to solve it [with AI]?" he said. "And do we have enough data to train a system? Can we get the data when we're ready to use [the system] in production, and can we actually get that data in enough real-time to be able to be useful to us?"
The data also need to be applicable to your institution, Hutson said.
"You may have a model being trained in California using California data, but is it applicable in New York?" he said. "It may or may not be."
Awareness of these AI issues will help institutions recognize if the technology is a good fit and avoid implementing a system that doesn't work for them, according to Hutson.
"We've all seen examples over the years of applications that may work in one context but certainly don't in another," he said. "Being able to understand how the underlying tools work is important in evaluating that."
The ultimate goal is data-driven decision-making, Hutson said.