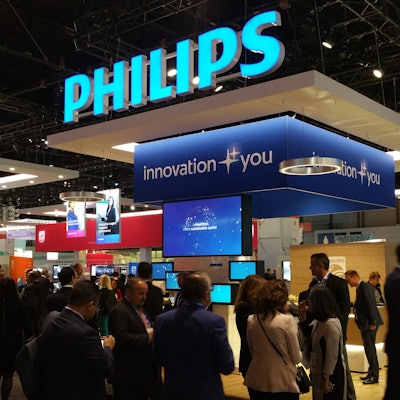
Philips Healthcare and Intel are reporting success from using computer central processing units (CPUs) to power deep-learning models for two radiology artificial intelligence (AI) applications.
Using Intel's Xeon scalable processors and the Open Visual Inference and Neural Network Optimization (OpenVINO) toolkit, the vendors found that a deep-learning model for predicting bone age on radiographs was 188 times faster than the initial baseline test. What's more, an algorithm for segmenting lungs on CT scans was 38 times faster, according to Intel.
The bone-age prediction algorithm improved from 1.42 images per second at baseline to a final tested rate of 267.1 images per second after optimizations, the company said. Meanwhile, the lung-segmentation model produced a processing rate of 71.7 images per second after optimizations, up from a baseline of 1.9 images per second and surpassing the target of 15 images per second, according to Intel.
The tests show that healthcare organizations can implement AI workloads without having to make expensive investments in hardware, Intel said.