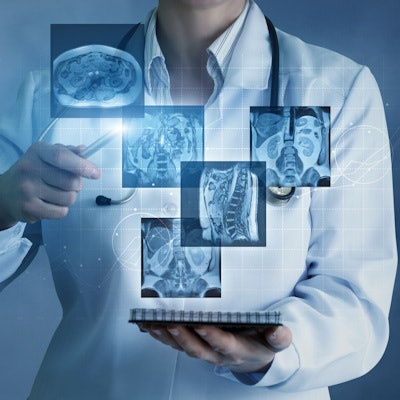
With virtual RSNA 2020 over and the year drawing to a close (finally!), I'd like to share my predictions about the world of radiology artificial intelligence (AI) for next year.
When all is said and done, 2020 was a big year for radiology AI, with even more algorithms cleared by the U.S. Food and Drug Administration (FDA) and the first-ever Medicare new technology add-on payment (NTAP) approval for AI in radiology.
In my opinion, the three major trends for 2021 that will take hold in the AI-medical imaging ecosystem are the following:
- AI will continue to be adopted by radiology departments and practices with a clearer return on investment (ROI).
- COVID-19 will accelerate the consolidation of radiology AI vendors.
- We will see a breakdown of AI into concrete categories, with algorithms no longer being lumped together under the broad term "AI."
Widespread AI and a clearer ROI
Unsurprisingly, my first prediction is that hospitals and private practices will continue to adopt radiology AI at an even faster pace than before. In a new study (Radiology: Artificial Intelligence, November 11, 2020, Vol. 2:6), Dr. Yasasvi Tadavarthi and colleagues estimated that next year the market cap for image analysis companies like Aidoc will hit a whopping $2 billion, up from $1.2 billion in 2019, due to more and more radiologists adopting AI into their workflow.
With over 30 FDA clearances issued to radiology AI this year alone and the recent announcement that a large vessel occlusion (LVO) prioritization algorithm will qualify for U.S. Centers for Medicare and Medicaid Services reimbursement under NTAP -- something that all AI vendors with similar functionality can take advantage of -- the return on investment from the usage of AI in clinical practice is becoming apparent.
But even with the advent of additional radiology AI use cases qualifying for NTAP, I don't believe there will be additional reimbursement avenues, and there will be more emphasis on understanding ROI prior to deployment in clinical practices.
COVID-19 changes the landscape -- consolidation and M&A
Even as radiology AI becomes more widespread than ever, 2022 will be a year of significant consolidation for developers. It is often said that the radiology AI market is an overhyped bubble, and COVID-19 might just put an end to that.
RSNA hosted 350 companies in 2019 in its "Machine Learning/Computer-Aided Diagnosis Systems" category. Given that the AI hype started more than half a decade ago, we can already see a divide between established players -- with regulatory clearances, active users, and recurring revenues -- and between new algorithm developers that will have to become more aggressive in their approach to market in order to catch up.
The numerous machine-learning companies that develop algorithms for image analysis are competing for an increasingly finite number of resources. COVID-19 has changed the playing field for everyone, especially the healthcare industry.
The combination of lower hospital budgets and reduced stability in the financial market will only widen the gap between industry players with significant adoption and those without. I believe we will see some radiology AI players begin to consolidate or liquidate, as well as potential mergers or acquisitions among the bigger players.
Breakdown of AI into concrete categories
Since the birth of the radiology AI industry, algorithms across all imaging modalities have been lumped together under the "AI" banner. Frankly, it made the AI section at RSNA look like a big mishmash of technologies.
It has always bothered me, since what we sell is value, not an underlying technology. As products mature with different use-cases, we will begin to see a focus on concrete categories of radiology AI, e.g., acute triage and notification, screening, communication, and population health. Companies will have a broad or narrow offering in each of those categories. We may even see conferences like RSNA opt to differentiate AI providers by types of solutions.
Elad Walach is a co-founder and CEO of Aidoc, a healthcare AI startup focused on using deep learning to relieve the bottleneck in medical image diagnosis. He is an expert in AI with visionary business insights in the healthcare space. Since founding Aidoc in early 2016, Elad has led the company through three investment rounds (raising over $60 million), driven the commercial availability of six FDA-cleared product lines, and created an install base of over 400 global hospitals while growing the company to over 120 employees. Elad began his career in the elite Israeli Defense Force technology program "Talpiot." He led AI research in the Israeli Air Force where he initiated and managed several teams focused on machine-learning and computer-vision projects. Elad holds a B.Sc. in Mathematics and Physics from The Hebrew University of Jerusalem and has published multiple academic papers on deep learning.