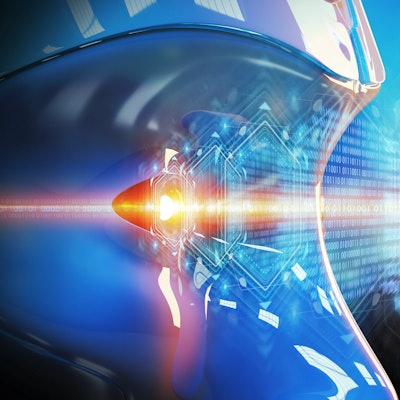
Suspicious liver lesions initially missed by radiologists on CT pulmonary angiography (CTPA) can be detected by an artificial intelligence (AI) model, according to research published September 17 in the Journal of the American College of Radiology.
Researchers led by Dr. Sarah Thomas of Duke University Medical Center used commercial AI software to retrospectively review one month of CTPA exams and reports from a teleradiology services provider. This approach to peer review enabled potentially missed cases of suspicious liver lesions to be identified more efficiently, requiring much less manual review of cases.
"Artificial intelligence can accelerate meaningful peer review by rapidly assessing thousands of examinations to identify potentially clinically significant errors," Thomas and colleagues wrote. "Although radiologist involvement is necessary, the amount of effort required after initial AI screening is dramatically reduced."
In an effort to test the feasibility of an AI-based model for expediting the peer-review process for incidental liver lesions on CTPA, the researchers first gathered 2,753 consecutive studies that were imaged at Virtual Radiologic in June 2017. These cases were retrospectively reviewed by two commercial applications from software developer CoRead AI, a proprietary software that visually classified the images for the presence of suspicious liver lesions that may require additional workup and then a natural language processing application to determine if the report mentioned a suspicious liver lesion.
Of the 2,573 CTPA studies, 136 were classified by the software as potentially containing missed suspicious liver lesions. Two abdominal radiologists, with three and four years of post-fellowship experience, respectively, then separately reviewed cases flagged by the software. Any discrepancies were resolved by a third reader with 11 years of post-fellowship experience.
Ultimately, 13 (0.5% of all cases) were confirmed as missed lesions.
"Using AI, the ratio of CTs requiring review to missed [suspicious liver lesions] identified was 10:1; the ratio without the help of AI would be at least 66:1," they wrote.
The researchers noted that the reviewing radiologists' interobserver agreement for suspicious liver lesions was excellent (k = 0.91).
"AI-augmented peer review can allow for the rapid and efficient review of more cases than feasible by human efforts alone, with relatively little direct radiologist effort," the authors wrote. "AI-assisted peer review also has the advantage of being blind to the initial reader, potentially removing the bias that comes with reviewing a colleague's cases."
The authors noted that AI review could be integrated into clinical workflows in a number of ways, including providing automated peer-review feedback after a case has been read or notifying the radiologist of a potential miss before they signed the report.
"Once 'missed' cases have been identified, this data combined with additional information such as scan parameters, radiologist experience, time spent reviewing the case, and time of day, could be leveraged to identify predictors of clinical errors," the authors wrote. "In the short term, AI quality assurance programs can provide valuable metrics on individual and group performance in specific areas, allowing for targeted educational interventions and tracking quality improvement initiatives over time."
Furthermore, AI could grow in the future to enable more efficient peer-review programs, according to the researchers.