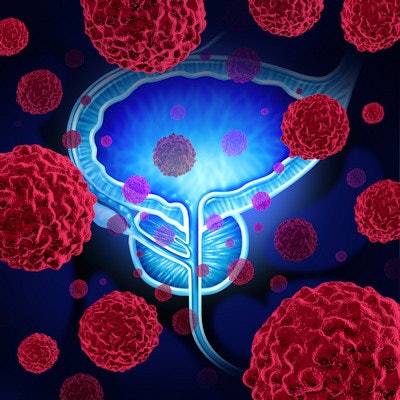
A deep-learning algorithm performed comparably to radiologist interpretations when it came to identifying prostate cancer on multiparametric MRI, according to study results presented at the RSNA 2021 meeting.
What's more, the combination of the algorithm with the radiologist was even more accurate than either alone. The findings could make multiparametric MRI for prostate cancer evaluation even more viable, said presenter Dr. Jason Cai of the Mayo Clinic in Rochester, MN, to session attendees.
"Prostate cancer is the second most common solid-organ malignancy in men, and multiparametric MRI helps guide diagnosis and treatment," he said. "It enables better patient selection for biopsy, facilitates the targeting of lesions during biopsy, and allows for tumor staging and monitoring treatment response."
Demand for multiparametric MRI for prostate cancer evaluation continues to increase, but image interpretation can be subject to reader variation, Cai noted. He and his team hypothesized that applying deep learning to multiparametric MRI could improve reader performance by offering support to radiologist readers.
Cai and colleagues developed a deep-learning pipeline that included segmentation of the prostate, preprocessing of the images, and classification of findings. The algorithm was trained on internal data gathered from all three Mayo Clinic sites (Minnesota, Florida, and Arizona); a cohort of 6,137 patients without history of clinically significant prostate cancer (Gleason grade groups 2 or higher) who underwent MRI between 2017 and 2019.
Of these patients, 1,594 were found to have clinically significant prostate cancer. The MRI exams included four sequences: T2; apparent diffusion coefficient (ADC); B1600 diffusion-weighted imaging (DWI); and T1 dynamic contrast-enhanced (DCE).
The internal dataset featured exams that had PI-RADS scores obtained from radiology reports. The team also used an external test set that included 204 exams taken from the publicly available ProstateX database. Four radiologists assigned PI-RADS scores to the ProstateX cases.
Cai's group used area under the receiver operating characteristic curve (AUC) and F1 scores to compare performance of the model and the radiologist readers and found that the deep-learning model performed comparably across a variety of measures.
Performance of deep-learning algorithm vs. radiologists for identifying prostate cancer on multiparametric MRI | |||
Measure | Radiologist reader | Deep-learning algorithm | Deep-learning algorithm and radiologist reader combined |
Internal dataset | |||
AUC | 0.89 | 0.90 | 0.94 |
F1 score | 0.75 | 0.78 | 0.84 |
External dataset (ProstateX) | |||
AUC | 0.84 | 0.86 | 0.88 |
F1 score | 0.71 | 0.73 | 0.78 |
"Lesion classification using PI-RADS is prone to intra- and interobserver variability, and a high level of expertise is required," Cai said. "In contrast, deep learning models produce deterministic outputs. We implemented a deep-learning model to detect clinically significant prostate cancer on multiparametric MRI, and we show that its performance is comparable to human readers on both internal and external test datasets. There is potential for our work to support radiologists in interpreting multiparametric MRI of prostate."