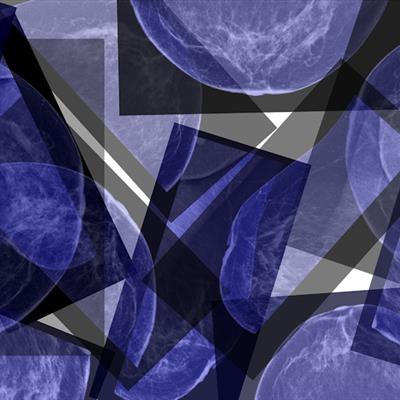
A deep-learning model created using data from a single breast MR image can help predict the five-year risk for women whose chances of developing breast cancer are higher, according to a study published in the July issue of the American Journal of Roentgenology.
The findings suggest yet another way breast cancer screening can be personalized in higher-risk women, wrote a team led by Tally Portnoi of the Massachusetts Institute of Technology in Cambridge.
"Accurate risk-assessment models can benefit high-risk patients, enabling more personalized screening strategies and preventive measures, including surgery and chemoprevention," the group wrote.
Although many models exist for evaluating breast cancer risk in the general population, models for women at higher risk are less available, the authors noted (AJR, July 2019, Vol. 213:1, pp. 227-233).
"Most of the existing models are well calibrated at the population level, but they are not sufficiently discriminative at the individual level," Portnoi and colleagues wrote. "This limitation becomes even more pronounced for high-risk cohorts, for whom commonly used risk factors are not sufficiently predictive and for whom it is less clear which additional patient features drive outcomes."
To address this gap, the group sought to develop a deep-learning model that could predict the five-year risk of breast cancer using a single breast MR image from a screening exam. The study included 1,656 consecutive contrast-enhanced screening breast MR images from exams performed for 1,183 high-risk women between January 2011 and June 2013.
The researchers compared the performance of the deep-learning model with another model they created using logistic regression based on traditional risk factors, as well as the Tyrer-Cuzick model, assessing all three with mean area under the receiver operating characteristic curve (AUC) values and observed-to-expected (OE) ratios.
The authors found that the deep-learning model based on the breast MR image had the highest AUC value. They also found that it was comparable to the Tyrer-Cuzick model when it came to OE ratios.
Performance of deep-learning model for breast cancer risk prediction | |||
Performance measure | Logistic regression model | Tyrer-Cuzick model | Deep-learning model based on breast MR image |
AUC | 0.56 | 0.49 | 0.64 |
Mean OE ratio | 0.83 | 1.09 | 0.99 |
The deep-learning approach is different from typical risk models, according to Portnoi's group.
"In contrast to existing models, relevant biomarkers are not explicitly identified; rather, the model learns useful features directly from the data," the researchers wrote. "This design enables the model to capture subtle patterns that may not be discernible to the human eye. ... [Also] the model can make predictions on the basis of an MR image only, without any knowledge of traditional risk factors required."
The study results are a promising beginning for the use of deep learning to personalize screening, according to the team.
"These results offer promising preliminary data regarding the potential of image-based risk-assessment models to support more personalized care," the group wrote.