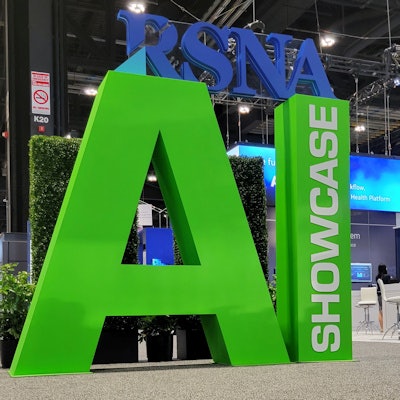
Artificial intelligence (AI) can reduce false-positive findings and potentially eliminate up to 80% of breast ultrasound exams from the radiologist worklist, according to research presented November 29 at the RSNA annual meeting.
A team led by Dr. Linda Moy from New York University trained their own AI model in their study of 45,000 exams, saying that hybrid decision-making models may enhance the performance of breast imagers without the added cost of a second human reader.
"Our AI system detects cancers exceeding that of board-certified radiologists and it may help reduce unnecessary biopsies and perhaps help harness enhance decision-making where there are shortages of radiologists," Moy said.
Ultrasound detects additional cancers when used as a supplemental screening method, but researchers said it has high false-positive rates, leading to unnecessary biopsies.
Moy and colleagues wanted to test the diagnostic accuracy of an AI model they developed, which they said automatically identifies malignant and benign breast lesions without requiring manual annotations from radiologists.
The AI system was trained using the university medical system's internal dataset of 288,767 ultrasound exams with 5,442,907 total images acquired from 143,203 patients between 2012 and 2019, including screening and diagnostic exams. Of these, 28,914 were associated with at least one biopsy procedure, 5,593 of which had biopsies yielding malignant findings.
The system was validated through a reader study with 10 board-certified breast radiologists. Each reader reviewed 663 exams that were sampled from the test set.
The team looked at a test set of 44,755 ultrasound exams and found that the AI system achieved an area under the curve (AUC) of 0.976 for identifying malignant lesions.
The system also had an AUC of 0.962 among the 663 reader study exams, significantly higher than the average radiologist, of which an AUC of 0.929 was achieved (p < 0.001). It also showed higher specificity than radiologists and recommended fewer biopsies.
Comparison between AI system and radiologists in interpreting breast ultrasound images | |||
AUC | Specificity | Biopsy recommendations | |
AI system | 0.962 | 85.6% | 19.8% |
Radiologists | 0.929 | 80.7% | 24.3% |
The hybrid models meanwhile improved the AUC for radiologists from 0.929 to 0.960. They also increased the average specificity for radiologists from 80.7% to 88.4% (p < 0.001) and their positive predictive value from 27.1% to 39.2% (p < 0.001). Their average biopsy rate was also decreased from 24.3% to 17.2% (p < 0.001).
"The reduction in biopsies using the hybrid models represented 29.4% of all recommended biopsies," the team wrote.
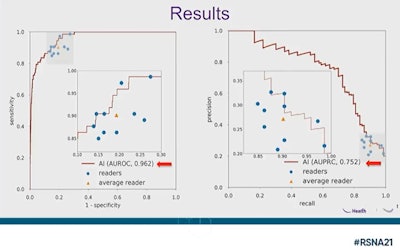
In the same test set of 44,775 exams, the group's AI system also had a false-negative rate of 0.008%, 0.02%, and 0.04% when triaging 60%, 70%, or 80% of women with the lowest AI scores.
When the AI system used a high specificity threshold to triage exams it considered to be at high risk for malignancy, it placed 2.2% of the total exams into an enhanced assessment workflow, with a positive predictive value of 69.6%, exceeding that of breast radiologists who initially evaluated the test set exams.
"Despite representing only 2.2% of the test dataset, this enhanced assessment workflow contained 56.5% of all malignant cases in the dataset," the researchers noted.
Because of this, Moy said AI-based software may serve as a standalone imaging interpreter. However, she added that additional studies addressing biases and larger datasets are needed.
"Adding clinical history and other information would hopefully make the models better readers and we can really see how they will work with clinical implementation," Moy said.