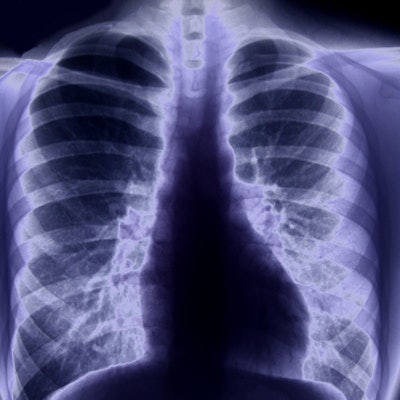
Artificial intelligence (AI) software can yield similar or even, by some measures, better performance than radiology residents in interpreting chest radiographs, and it could potentially be utilized for automated preliminary assessments of these exams, according to research published online October 9 in JAMA Network Open.
Researchers led by first author Dr. Joy Wu and senior author Tanveer Syeda-Mahmood, PhD, of IBM Research compared the performance of a deep-learning model with that of five third-year radiology residents on nearly 2,000 chest radiographs from emergency departments (EDs). The team found that the algorithm yielded similar sensitivity but statistically higher specificity and positive predictive value, compared with the residents.
"Integrating such AI systems in radiology workflows for preliminary interpretations has the potential to expedite existing radiology workflows and address resource scarcity while improving overall accuracy and reducing the cost of care," the authors wrote.
Using a training dataset of 342,126 frontal chest radiographs acquired in ED and urgent care settings, the team of researchers trained an algorithm to assess the studies for the presence of 72 findings they considered to represent a full-fledged preliminary read.
The researchers then selected five third-year radiology residents from academic medical centers around the U.S. after they had passed a reading adequacy test. Blinded to the AI algorithm estimates, these residents each interpreted approximately 400 nonoverlapping sets of anteroposterior (AP) frontal chest radiographs from a hospital source.
In comparison with the ground truth, the algorithm yielded a pooled k value of 0.544 on a per-finding basis, while the residents had slightly higher agreement -- producing a pooled k value of 0.585.
"In general, residents performed better for more subtle anomalies, such as masses and nodules, misplaced lines and tubes, and various forms of consolidation, while the AI algorithm was better at detecting nonanomalous findings, the presence of tubes and lines, and clearly visible anomalies, such as cardiomegaly, pleural effusion, and pulmonary edema," the authors wrote. "Conversely, the AI algorithm generally performed worse for lower-prevalence findings that also had a higher level of difficulty of interpretation, such as masses or nodules and enlarged hilum."
The researchers also assessed preliminary interpretation performance by comparing results on a per-image basis.
AI vs. residents for preliminary interpretation of chest radiographs | ||
Radiology residents | AI algorithm | |
Mean image-based sensitivity | 72% | 71.6% |
Mean image-based positive predictive value | 68.2% | 73% |
Mean image-based specificity | 97.3% | 98% |
"These findings suggest that it is possible to build AI algorithms that reach and exceed the mean level of performance of third-year radiology residents for full-fledged preliminary read of AP frontal chest radiographs," Wu and colleagues wrote. "This diagnostic study also found that while the more complex findings would still benefit from expert overreads, the performance of AI algorithms was associated with the amount of data available for training rather than the level of difficulty of interpretation of the finding."
Even if the AI software is utilized to perform preliminary interpretations to target the most prevalent findings, final reads should still be performed by the attending physician, however, to avoid potential misses of less-common results, according to the researchers.
"Having attending physicians quickly correct the automatically produced reads, we can expect to significantly expedite current dictation-driven radiology workflows, improve accuracy, and ultimately reduce the overall cost of care," the authors wrote.