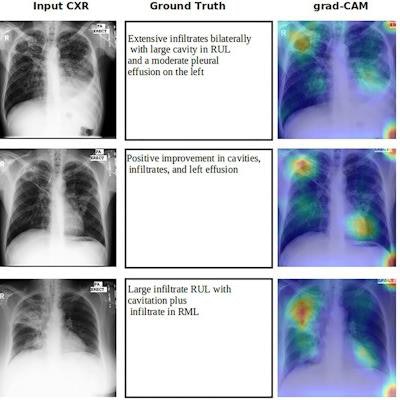
Researchers in India have developed a new artificial intelligence (AI) model for detecting active tuberculosis on chest x-rays, and they suggest that the approach offers significant potential for real-world applications in locations with limited resources.
A group led by James Devasia at the Jawaharlal Institute of Postgraduate Medical Education and Research in Pondicherry developed a deep-learning AI model that identifies tuberculosis based on 44 lung abnormalities. The model performed well identifying the varied manifestations of tuberculosis, according to the authors.
"This work is one of the first attempts in the multilabel classification of the manifestations of active [tuberculosis] with potential to widen the possibility of real-world application in primary care settings with limited resources," the group wrote. The study was published January 17 in Scientific Reports.
Chest x-ray is the oldest and primary radiologic method for detecting tuberculosis. In developing countries with a high burden of the disease, the lack of trained clinicians in primary care settings has spurred efforts to use AI to fill the gap.
In this study, the authors fine-tuned a deep neural network called EfficientNet B4 for multilabel classification of specific tuberculosis abnormalities. Using a dataset of 1,312 portable chest x-rays from 837 patients, they trained the neural network to classify 30 abnormalities in the upper, middle, and lower zones of the left and right lung, and 14 abnormalities in left and right lungs.
They used 36 abnormal chest x-rays curated from a public dataset maintained by the U.S. National Library of Medicine to validate the model, while the ground truth was established by a trained radiologist who examined all images.
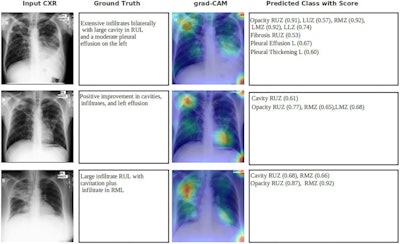
The trained model achieved "remarkable" area under the receiver operating characteristic (AUC), sensitivity, and specificity for detecting various pulmonary tuberculosis manifestations, the authors wrote. Specifically, the model achieved AUC ranges from 0.95 to 1 with calcification showing the lowest AUC and pleural effusion showing the highest. Sensitivity ranged from 70% to 100%, and specificity ranged from 50% to 100%.
"EfficientNet B4 trained network achieved remarkable AUC, sensitivity, and specificity of various pulmonary tuberculosis manifestations in intramural test set and external test set from different geographical regions," the authors wrote.
Finally, the researchers visualized the model's potential performance in a clinical setting by using a gradient-weighted class activation mapping (Grad-CAM) method, which generated heat maps showing the manifestations of active tuberculosis in affected lung areas.
"This localization heatmap is remarkable for its meticulousness and can be used in clinical settings to aid the clinicians in primary health care where service from expert radiologists is often lacking or nil," the authors wrote.
Ultimately, in primary care clinical settings, it is crucial to identify tuberculosis abnormalities in chest x-rays that are distinguishable from other thoracic diseases for early diagnosis, and this study points in a new direction for AI development, the authors noted.
"Further research in the classification of various abnormalities of active tuberculosis is necessary and there is a scarcity of literature in this domain," Devasia and colleagues concluded.