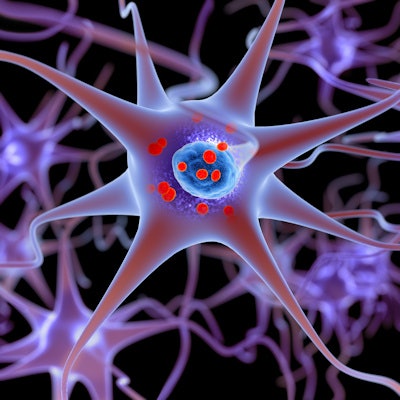
An artificial intelligence (AI) algorithm was highly accurate for diagnosing Parkinson's disease on dopamine transporter (DaT) SPECT exams, according to research presented at the annual meeting of the Society of Nuclear Medicine and Molecular Imaging (SNMMI).
Using an approach that relies only on the raw projection data in DaT SPECT exams, a deep learning-based technique developed by researchers from Johns Hopkins University in Baltimore yielded 97% accuracy for identifying Parkinson's disease on a test set of cases. The 3D convolutional neural network (CNN) showed significant promise for correctly classifying subjects with Parkinson's disease as well as healthy patients, according to presenter Kevin Leung, PhD.
DaT SPECT is an established diagnostic tool that's routinely used for diagnosis of Parkinson's disease. However, the standard clinical practice of performing visual analysis of images along with striatal DaT quantification is suboptimal due to interobserver variability, Leung said.
Also, DaT-SPECT images that are reconstructed by iterative methods such as ordered subset expectation maximization (OSEM) are strongly affected by reconstruction parameters. In addition, heavy postreconstruction smoothing techniques are often applied in the clinic in order to reduce noise in images, he said.
"Due to all of these processes, some information present in the original projection data could be lost in reconstructed images," Leung said.
Hypothesizing that there might be more information present in the projection data that's important for disease detection and classification tasks, the researchers sought to train a 3D CNN algorithm to identify Parkinson's disease in patients using just the DaT SPECT projection data.
They gathered 659 DaT-SPECT image volumes from healthy controls and patients with Parkinson's disease in the open-source Parkinson's Progression Markers Initiative (PPMI) database. An analytical projector that incorporated attenuation and spatially varying resolution was used to generate projection data from the DaT-SPECT volumes, according to the researchers.
Of the 659 cases, 80% were used for training and 10% were utilized for validation. The remaining 10% were set aside for testing. The CNN architecture included a series of 3D strided convolutional layers, followed by a global average pooling layer and then a fully connected layer, according to Leung.
The DaT-SPECT 3D projection data were used as inputs for the CNN, which then outputs a predictive likelihood of whether the patient was healthy or had Parkinson's disease, according to the researchers.
AI performance for diagnosing Parkinson's disease on test set of DaT-SPECT cases | |
3D CNN model | |
Sensitivity | 98% |
Specificity | 95% |
Accuracy | 97% |
Area under the curve | 0.96 |
The results demonstrate the accurate prediction performance for the deep-learning method in Parkinson's disease, according to the researchers.
"Future work will compare the performance of this approach with networks that detect Parkinson's disease in reconstructed SPECT images," Leung said.