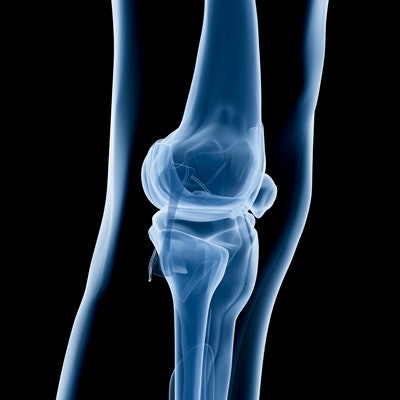
A deep-learning algorithm can accurately segment knee MRI scans and extract quantitative data in seconds, potentially helping radiologists to easily provide clinicians with valuable data for diagnosing, assessing, and treating osteoarthritis (OA), according to a team from the University of California, San Francisco (UCSF).
The UCSF group has developed a neural network that fully segments cartilage and meniscus compartments on both high-resolution and low-resolution MR images and then extracts morphometry and relaxometry data. Although the process takes less than 10 seconds, the algorithm yields results that correlate well with those produced by more time-consuming manual and semiautomatic segmentation methods, said Berk Norman, a research data analyst at UCSF's Musculoskeletal Quantitative Imaging Research lab.
"It accurately extracts morphologic volumes and relaxation times in regions of challenging compartmental differentiation," said Norman. He presented the UCSF work at the recent Society for Imaging Informatics in Medicine's Conference on Machine Intelligence in Medical Imaging (C-MIMI 2017) in Baltimore.
Osteoarthritis
OA is a degenerative disease that's on the rise in the aging population; in the U.S., the condition has been conservatively estimated to affect 13.9% of adults older than 25 and 33.6% (12.4 million people) of those older than 65, Norman said. The disease reduces quality of life and can cause significant pain and discomfort.
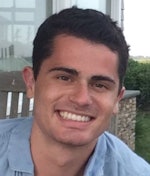
Quantitative MRI can be used to diagnose, assess, and monitor diseases such as osteoarthritis by identifying morphologic changes in the knee and calculating T1 rho and T2 relaxation times, he said. However, the clinical application of quantitative MRI has been hindered by the requirement for time-consuming postprocessing of images, particularly for segmentation of the joint and musculoskeletal tissue.
Currently, the segmentation required for quantitative MRI is performed either manually or semiautomatically.
"If it's done manually, it can take a skilled technician around three hours to do an image, depending on the quality of the image and what actually is being segmented," Norman said. "Additionally, manual and semiautomatic methods are subject to inter- and intrauser variability that can make reproducibility difficult across studies."
As a result, the UCSF team sought to utilize neural networks to develop a fully automatic segmentation algorithm that could accurately extract cartilage volume and thickness measurements as well as T1 rho and T2 relaxation times on knee MRI. Four cartilage compartments and two meniscus compartments are segmented by the algorithm.
Training and testing
The algorithm was developed using two datasets. To train and test the algorithm to extract T1 rho and T2 relaxation times, the researchers used a dataset of T1-weighted image volumes from 415 patients: 85 patients with osteoarthritis, 115 patients with an anterior cruciate ligament (ACL) injury, and 215 control subjects. They included control subjects and ACL injury cases to make sure the deep-learning model would yield consistent performance across different patient cohorts, Norman said.
Of the 415 image volumes, 332 (6,970 slices) were used for training and 83 (1,746 slices) were reserved for testing.
"This was a relatively small dataset size, so we decided to do training and testing on a per-slice basis, which increased our training set size to around 7,000 slices and in testing to around 2,000 slices," he said. "This also allowed us to do 2D modeling, avoiding some of the computational issues that come with 3D models."
All cases in the dataset used a spoiled gradient-recalled echo (SPGR) T1-weighted MRI sequence, including a repetition time/echo time (TR/TE) of 9/2.6 msec, a 1,500-msec time of recovery, a 14-cm field-of-view, a 256 x 128 matrix, a 4-mm slice thickness, and a 62.5-kHz bandwidth.
Next, the team trained the algorithm to extract cartilage volume and thickness measurements by using a dataset of 3D double-echo steady-state (DESS) MR sequences from 174 patients, which included 113 patients with OA and 215 control subjects. Of these 174 high-resolution image volumes, 135 (21,440 slices) were used for training, while 38 (6,080 slices) were set aside for testing.
The 3D-DESS sequences included a 4.7-msec TE, a 16.2-msec time of recovery, a 14-cm field-of-view, a 307 x 384 matrix, a 0.7-mm slice thickness, and a 185-kHz bandwidth.
An end-to-end method
Most current automatic or semiautomatic segmentation methods are based on an atlas- or patch-based approach that requires costly preprocessing and postprocessing, Norman said. As a result, the UCSF group elected to go with a 2D U-Net convolutional neural network, an end-to-end method that doesn't require preprocessing of images. Notably, the researchers also elected to employ a deep-learning approach called weighted cross-entropy, which enabled the algorithm to learn faster and more accurately, he said.
After the algorithm was trained and tested, the researchers used Dice coefficients to compare the model's effectiveness in relation to the results achieved by manual segmentation. They also assessed the effectiveness of both methods for quantifying morphology and extracting T1 rho and T2 relaxation times.
The algorithm yielded comparable segmentation performance to manual segmentation, according to the researchers.
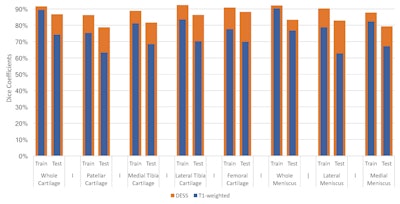
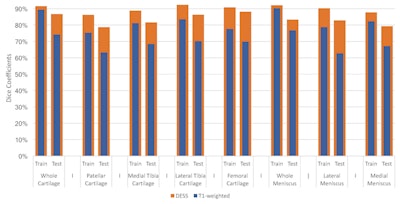
Dice coefficient chart compares the algorithm's training and testing performance for extracting morphometry on DESS images (orange bars) and for extracting T1 rho and T2 relaxation times on T1-weighted images (blue bars) with results produced by manual segmentations. Image courtesy of Berk Norman.
"These are very strong Dice coefficients that are [comparable to] or even outperform some of the state-of-the-art semiautomatic and automatic segmentation methods currently being used for knee multitissue segmentation," he said.
In other findings, the analysis showed that the algorithm's performance -- in comparison with manual segmentation -- was better on the DESS images than on the T1 images for all cartilage and meniscus compartments. This wasn't too surprising, however, as DESS is a higher-quality image that may provide more features for the algorithm to learn from and extract better segmentations, Norman said.
"Initially we had less overall data from the T1-weighted dataset compared with the DESS dataset," he said. "This also may explain why there's a slightly larger discrepancy between the training and testing [performance] for the T1-weighted set compared to the difference in training and testing for the DESS dataset."
Relaxometry
The algorithm's calculation of T1 rho and T2 relaxation times on the T1-weighted data correlated highly with those compiled using manual segmentation, according to the group.
Absolute mean difference in T1 rho segmentation relaxation time compared with manual segmentation | ||
Model on training cases | Model on testing cases | |
Femoral cartilage | 0.2676 msec | 0.1169 msec |
Lateral tibial cartilage | 0.5951 msec | 0.5392 msec |
Medial tibial cartilage | 0.0627 msec | 0.1326 msec |
Patellar cartilage | 0.0258 msec | 0.4334 msec |
Absolute mean difference in T2 segmentation relaxation time compared with manual segmentation | ||
Model on training cases | Model on testing cases | |
Femoral cartilage | 0.2907 msec | 0.2632 msec |
Lateral tibial cartilage | 0.4398 msec | 0.5647 msec |
Medial tibial cartilage | 0.1531 msec | 0.0946 msec |
Patellar cartilage | 0.4116 msec | 0.4005 msec |
"There was no statistically significant difference between [the algorithm and manual segmentation]," he said.
Morphometry
The algorithm also correlated highly with manual segmentation for calculating cartilage thickness and volume.
Absolute mean difference in cartilage thickness measurements compared with manual segmentation | ||
Model on training cases | Model on testing cases | |
Femoral cartilage | 0.2051 mm | 0.1952 mm |
Lateral tibial cartilage | 0.0874 mm | 0.0681 mm |
Medial tibial cartilage | 0.1531 mm | 0.0946 mm |
Patellar cartilage | 0.4116 mm | 0.4005 mm |
Absolute mean difference in cartilage volume measurements compared with manual segmentation | ||
Model on training cases | Model on testing cases | |
Femoral cartilage | 1479.7 mm3 | 1122.4 mm3 |
Lateral tibial cartilage | 107.78 mm3 | 58.28 mm3 |
Medial tibial cartilage | 231.64 mm3 | 104.72 mm3 |
Patellar cartilage | 614.99 mm3 | 436.22 mm3 |
The only statistically significant differences between the algorithm and manual segmentation in morphometry were found in the testing cases for calculating the thickness of the patellar cartilage and the femoral cartilage, Norman said.
"After looking in to this, [we found that the two methods] still had a very strong linear relationship," he said. "The only difference between the two is that the [automatic] segmentation method was just slightly overestimating the thickness, but this could be adjusted using a bias correction."
A speedier approach
What's more, the researchers' method was much faster than manual or semiautomatic segmentation approaches, which can take up to three hours to perform.
"Our method only takes about 2.5 seconds to reconstruct a low-resolution volume and eight seconds to reconstruct a high-resolution segmentation mask," Norman said.
In addition, the algorithm yielded invariant results across control, OA, and ACL injury patients. This means it has potential for use in all patient cohorts, according to the group.