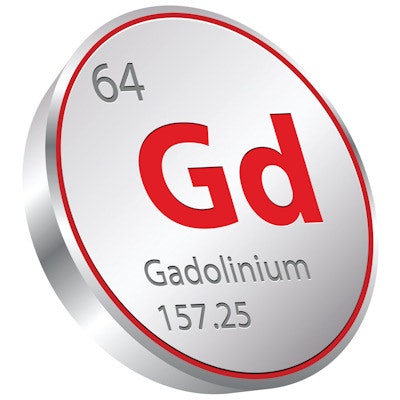
Researchers developed an artificial intelligence (AI) algorithm that accurately detected chronic myocardial infarction (MI) on noncontrast-enhanced cardiac cine MRI, potentially obviating the need for gadolinium-based contrast agents in these patients, according to research published online April 30 in Radiology.
A team of researchers led by Dr. Nan Zhang of Capital Medical University in Beijing and Guang Yang of the Royal Brompton Hospital and the Imperial College London in the U.K. reported that their deep-learning algorithm was highly sensitive and specific for diagnosing chronic MI. They also found no statistical difference between the areas of chronic MI detected by the algorithm on noncontrast-enhanced MRI and manual segmentations performed by an expert radiologist on gadolinium-enhanced MRI.
Late gadolinium enhancement on MRI scans has become the ground-truth reference technique for cardiac MRI, the researchers noted. But gadolinium is contraindicated in patients with severe renal impairment, and concerns about gadolinium deposition in patient tissue have sparked interest in finding ways to reduce or replace gadolinium where possible.
In the current paper, researchers discussed the use of an algorithm they developed that could help. The algorithm is based on a recurrent neural network and first extracts representative local and global motion features from nonenhanced cardiac cine MRI. It can then predict the location, size, and transmurality of MI without gadolinium-enhanced imaging, according to the researchers.
The group trained and tested an algorithm on 212 patients with chronic MI and 87 healthy controls who had all received both nonenhanced cardiac cine MRI (25 phases per cardiac cycle) and late gadolinium-enhanced MRI. Of the participants, 80% were used to train the model and 20% were utilized for testing.
Performance of deep-learning model for detecting chronic myocardial infarction | |
Deep-learning model | |
Sensitivity | 89.8% |
Specificity | 99.1% |
Area under the curve | 0.94 |
In comparison with the ground-truth manual segmentations performed by a radiologist with seven years of experience in cardiovascular MRI, the deep-learning algorithm didn't produce statistically significant differences in calculating the number of MI segments, per-patient MI area, and MI area percentage.
The researchers said that their deep-learning framework can be readily expanded for use in future prospective studies.
"Future larger-scale studies with data from multiple sites are required for a full validation of our deep-learning framework," they wrote. "These would also allow the accuracy of MI prediction to be determined for different myocardial segments with different motion characteristics. Further comparison with microvascular obstructions excluding data and texture analysis will be investigated in future work."
In an accompanying editorial, Dr. Tim Leiner, PhD, of Utrecht University Medical Center in the Netherlands noted that cardiac MRI without the use of gadolinium-based MRI contrast isn't yet clinically feasible, as sufficient evidence from prospective multicenter outcome studies doesn't currently exist.
"Until these data are available, cardiac imagers will keep injecting gadolinium-based contrast agent, or they will select alternative imaging modalities in patients for whom contrast-enhanced cardiac MRI is contraindicated," he wrote.
He congratulated the authors, though, for demonstrating the promise of deep learning in assessing the presence and extent of myocardial scar tissue in patients with chronic MI.
"Their work is another important step forward in making cardiac MRI safer, faster, more cost-effective, and more patient friendly," Leiner wrote.