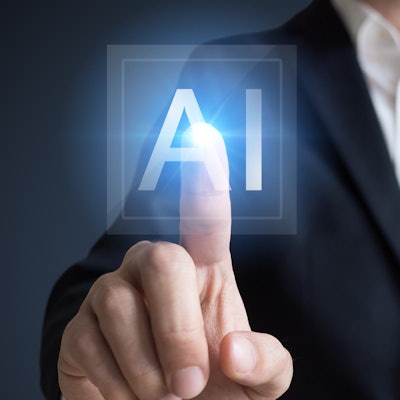
Artificial intelligence (AI)-based analysis of neuroimaging and neurocognitive measures shows promise as a tool for diagnosing bipolar disorder, according to a small proof-of-concept study published online February 26 in the Asian Journal of Psychiatry.
A group of researchers led by Dr. Rashmin Achalia of Government Medical College in Aurangabad, India, found that a machine-learning algorithm achieved the highest accuracy for bipolar disorder when it took into account neuroimaging -- structural MRI, resting-state functional MRI, and diffusion tensor imaging (DTI) -- along with neuropsychological test results.
"The findings of the study provide proof-of-concept evidence for the development of objective biomarkers for individuals with [bipolar disorder]," the authors wrote.
Although the combination of multimodal imaging measures and neurocognitive measures has been shown in the literature to be highly accurate for classification of Alzheimer's disease, the combination of these techniques using machine learning has not yet been explored for the task of differentiating patients with bipolar disease from healthy subjects, according to the researchers.
Seeking to test their hypothesis that the composite analysis of both measures would yield better classification accuracy than the individual measures would achieve on their own, the researchers recruited 30 patients with type I bipolar disorder among those attending the outpatient psychiatry clinic at the Government Medical College. They also signed on 30 age- and sex-matched healthy volunteers from the same area.
Of these 60 participants, 50 -- 25 healthy volunteers and 25 bipolar disorder patients -- served as the training set. The remaining 10 participants were utilized as the testing set.
The participants all received structural MRI, resting-state functional MRI, and DTI on a 1.5-tesla scanner. In addition, they were all given neuropsychological tests by an experienced clinical psychologist in order to assess executive functioning.
The authors said that support vector machine (SVM) machine-learning classifiers for each modality were trained to compare and analyze the available data to ascertain the best biomarkers for bipolar disorder. Next, the most useful features from all modalities were taken together as an input to train a composite algorithm. The feature combinations for individual modalities and for the composite model were then validated with k-fold cross-validation over 10 trials, according to the researchers.
The researchers found that the algorithm trained using the combination of neuroimaging and neurocognitive data yielded the best performance.
Performance of machine-learning algorithm for diagnosing bipolar disorder | |||
Sensitivity | Specificity | Accuracy | |
Machine-learning algorithm | 82.3% | 92.7% | 87.6% |
"While individual modalities provided accuracy levels ranging between 70% and 80%, the combination of multiple modalities provided the best accuracy of 87%, which is important in clinical applications," the authors wrote. "Our composite five-factor model exceeded the accuracy threshold for markers, >80% sensitivity and >80% specificity, required for neuropsychiatric conditions."
The researchers believe this approach could eventually provide an objective marker to aid in the diagnosis of bipolar disorder.
"Findings of this proof-of-concept study, if replicated in larger samples, could have potential clinical applications," they wrote.