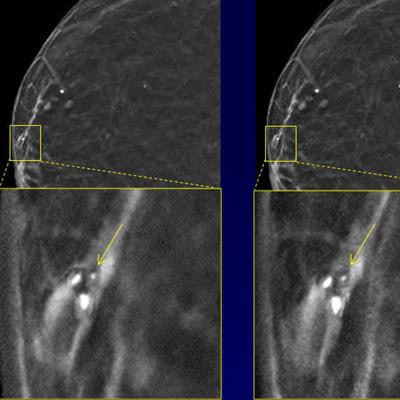
An artificial intelligence (AI) algorithm may enable breast imagers to sharply reduce radiation dose from digital breast tomosynthesis (DBT) exams -- perhaps by as much as 80%, according to research presented last month at the SPIE Medical Imaging 2018 conference in Houston.
A multi-institutional team led by Junchi Liu from the Illinois Institute of Technology in Chicago developed a deep learning-based image processing technique that can convert lower-dose DBT images to virtual images that approximate higher-dose exams. In testing on images acquired on cadaver phantoms, the method yielded high-quality images at as little as 79% of the dose of standard DBT studies.
What's more, an observer study involving 10 breast radiologists and 10 clinical DBT cases found no statistically significant difference in quality between real full-dose images and the virtual high-dose images produced by the deep-learning algorithm from half-dose images.
"Our [technique] converts [low-dose] images to 'virtual' [high-dose] images where noise and artifact in the [low-dose] images are significantly reduced, while maintaining breast tissue and subtle structures such as tiny microcalcifications," Liu told AuntMinnie.com. "To our knowledge, there is no [other] deep-learning techniquethat has been developed for radiation dose reduction in DBT."
The research was presented at the SPIE Medical Imaging 2018 meeting by senior author Kenji Suzuki, PhD, also from the Illinois Institute of Technology.
DBT advantages, disadvantages
DBT has been found to be a promising modality for detecting breast cancer, offering higher cancer detection rates and fewer false positives than traditional 2D mammography. However, these benefits come at the cost of higher radiation exposure; the two DBT clinical scenarios approved by the U.S. Food and Drug Administration (FDA) could deliver a 1.4 to 2.3 times higher radiation dose than mammography, Liu said.
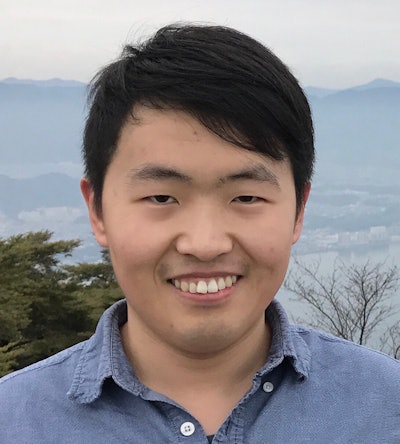
As a result, repeat DBT for annual screening could increase cumulative radiation exposure and the lifetime risk for radiation-induced breast cancer, according to the group, which also included Dr. Laurie Fajardo from the University of Utah in Salt Lake City, as well as researchers from the University of Iowa Hospitals and Clinics in Iowa City. Although noise reduction techniques may be used to reduce radiation dose in DBT, it's challenging to reduce noise and artifacts on the images while maintaining important diagnostic information and the depiction of subtle lesions.
To address this issue, the group developed a supervised image-processing technique based on a deep-learning method called neural network convolution (NNC). Although NNC and the commonly used convolutional neural network (CNN) are both deep-learning methods, they differ significantly in terms of their architecture, output, and required numbers of training samples. In CNNs, convolutional operations are performed within the network; convolutional operations are performed outside of the network in NNCs.
Furthermore, CNNs typically output class categories, while the NNCs output images. Also, CNNs require a large number of training images due to the large number of parameters in the model. NNCs, on the other hand, need only a very small number of training images, Liu said.
"In contrast to typical deep-learning techniques that output class labels, NNC is able to directly learn and output desired images," he said.
The group's patch-based technique converts lower-dose DBT images to virtual higher-dose images, reducing noise and artifacts in the lower-dose images while maintaining breast tissue and subtle structures, according to the researchers. The group trained and validated the deep-learning algorithm using quarter-dose (12 mAs at 32 kVp) raw projection images and corresponding "teaching" images acquired at 200% of the standard dose (99 mAs at 32 kVp) from breast cadaver phantoms. All images were acquired using a Selenia Dimensions DBT system (Hologic).
After training, the algorithm no longer needs actual high-dose images to produce its virtual high-dose images. Using NVIDIA's GeForce GTX Titan Z graphics processing unit (GPU) on an ordinary PC powered by an Intel i7-4790K central processing unit operating at 4 GHz, the algorithm took 0.24 seconds for the method to process each study. Processing time without the GPU took 3.6 seconds.
The team then evaluated the image quality of the phantom images using the structural similarity (SSIM) index; the higher the SSIM index, the more similar the two images being compared. The deep-learning algorithm was able to convert quarter-dose images (1.35 mGy; SSIM: 0.88) to virtual higher-dose images with image quality (SSIM: 0.97) that was equivalent to images at 119% of dose (6.41 mGy). This represents a 79% dose reduction, according to the group. The technique also yielded higher SSIM than three other well-known DBT noise reduction methods: bilateral filtering, block-matching and 3D filtering (BM3D), and K-SVD.
Clinical cases
To further evaluate the robustness and performance of the technology, the researchers applied their trained NNC model to 10 clinical screening cases. In an observer study involving 10 breast radiologists at the University of Iowa Hospitals and Clinics, the technology provided full-dose equivalent images from half-dose images.
"It reduced noise in half-dose images while preserving calcifications and breast tissue structures," Liu said.
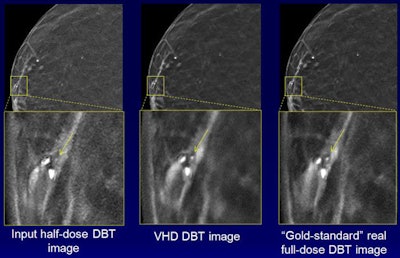
Seven (70%) of 10 breast radiologists either preferred the virtual higher-dose DBT images over the real full-dose images or could not distinguish between the two, according to the group. The difference in image quality between the image types wasn't statistically significant (p = 0.59).
"In other words, [virtual high-dose] DBT images and real full-dose DBT images are equivalent, thus achieving 50% dose reduction," Liu said. "Our technology is in the stage of preclinical use. We plan to perform a bigger observer rating study with more radiologists. Once it is done, our technology is ready for clinical use following FDA approval."