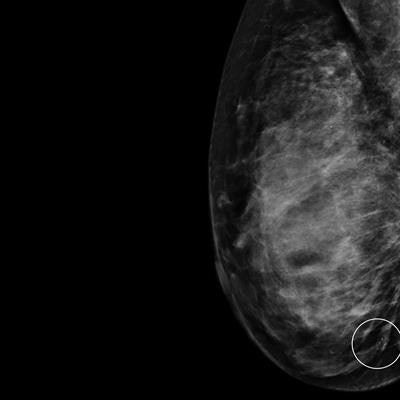
Radiologists interpreting mammograms demonstrated a wide range of sensitivity for detecting cancer, according to results of a study published July 28 in Radiology. The authors believe the findings justify the use of computer-aided detection (CAD) solutions for breast screening.
In the study, Swedish researchers compared the performance of radiologists who interpreted screening cases in a cohort of 1,186,045 mammograms, classifying performance by tumor subtype. The team found that mean sensitivity ranged from 63% for those in the lowest quartile to 84% for those in the most sensitive quartile. Specificity ranged from 95% to 98%.
The sensitivity difference was very large for basal cancers, with the least sensitive and most sensitive high-volume readers detecting 53% and 89% of cancers, respectively (p < 0.001).
"Screening mammography performance benchmarks are an important metric upon which radiologist practice is measured. The ongoing development of artificial intelligence (AI) CAD systems will require comparison to radiologist performance to prove effectiveness and thus such benchmarks are important," first author and radiology resident Dr. Mattie Salim from the Karolinska Institute told AuntMinnie.com.
The mammograms were performed at five different institutes between 2008 and 2015 on 418,041 women ages 40 to 74 in Stockholm county who were invited to be screened every 18 to 24 months. Of the 110 interpreting radiologists, 24 were deemed high-volume readers (those who performed over 5,000 annual screening mammograms), and these high-volume readers interpreted 972,889 mammograms from the total cohort. The 86 low-volume readers interpreted the remaining 213,146 mammograms.
Among women who underwent screening, 4,723 were diagnosed with breast cancer at screening or within 12 months thereafter. There were 3,514 true-positive screenings, 1,209 false-negative screenings, 1,138,619 true-negative screenings, and 41,969 false-positive screenings. The mean age at screening was 54 ± 9.5 (standard deviation), and the mean age at diagnosis was 59 ± 10.1. Women were considered to have had breast cancer at the time of screening if the breast cancer quality registry indicated the diagnosis within 12 months after the mammogram had been obtained.
The researchers then assessed reader sensitivity, and divided the variation by quartiles, from least to most sensitive. For high-volume readers, the performance was as follows:
Variation in reader performance of screening mammograms, by quartile | ||||
Quartile 1 | Quartile 2 | Quartile 3 | Quartile 4 | |
Sensitivity | 63% | 68% | 78% | 84% |
Specificity | 95% | 96% | 97% | 98% |
Tumor subgroups
Researchers also analyzed sensitivity by tumor types. The overall sensitivity for high-volume readers was 77% for ductal cancers and 73% for lobular cancers, 76% for invasive cancers and 83% for in situ only (any grade) cancers, 77% for luminal A, and 69% for basal cancers.
Analysis by quartile of high-volume readers showed that the mean sensitivities for the most (Q4) and the least (Q1) sensitive high-volume readers were 85% and 67%, respectively, for ductal cancers; 84% and 63%, respectively, for lobular cancers; 85% and 67%, respectively, for all invasive cancers; 93% and 75%, respectively, for in situ cancers only (any grade); 99% and 75%, respectively, for high-grade in situ cancers only; 85% and 67%, respectively, for luminal A cancers; and 89% and 53%, respectively, for basal cancers (p < 0.001 for the comparison of sensitivity levels between quartiles for each of the subgroups).
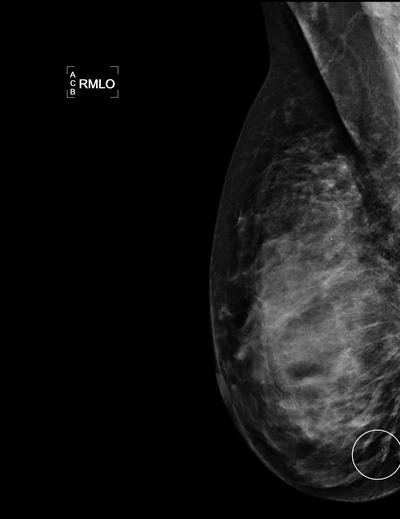
"Besides the wide range of performance between high-volume readers where the mean sensitivity per quartile ranged between 63% and 84%, we also find the results for tumor characteristics quite interesting whereby the largest performance difference was observed for basal molecular subtype with sensitivity ranging between 53% to 89%. Both results are important when evaluating the performance of AI CAD systems," Salim noted.
The authors hope that the paper will be useful when choosing the operating point of standalone AI CAD systems and that it will be used as guidance for future evaluations making it easier to implement AI systems in daily practice.
"Applying AI cancer detection software could potentially reduce the wide variation in sensitivity that the screening participants experienced," she said.
Under principal investigator Dr. Frederik Strand, the team will soon present results from a new study that directly compares the performance of three commercially available AI algorithms and radiologists in screening mammography.
Commenting on the paper, Dr. László Tabár, professor of radiology from Falun, Sweden suggested that using the mammographic appearance of the cancers -- rather than histology -- would be more informative to describe the cancers that are easy to find versus those most often missed.
Tabár, who was not involved in the research, cited stellate/spiculated cancers and microcalcifications as being among those detected with a higher sensitivity, while circular/oval-shaped cancers as well as architectural distortion are, although often fast-growing, aggressive cancers, frequently missed.
"Before one evaluates AI CAD as a potential helper in screening, I would recommend improving and standardizing the viewing technique of each screening mammogram, which can be described as follows: 'synchronous enlargement of both mammograms followed by side-by-side step-by-step viewing.' I am positive that the sensitivity figures of readers could significantly benefit from it, setting a bigger challenge for the combined AI CAD," Tabár noted.