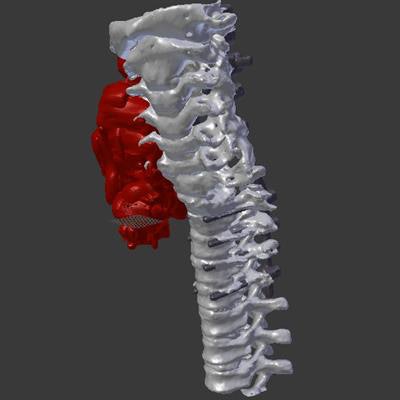
How can artificial intelligence (AI) help ameliorate the high cost and long turnaround time of producing 3D-printed models? One possibility is by automating steps in the 3D printing process, according to a review article published online May 9 in Expert Review of Medical Devices.
The researchers, led by senior author Dr. Jorge Zuniga from the University of Nebraska in Omaha, reviewed dozens of publications related to the integration of machine learning and 3D printing. They found that several studies demonstrated the potential of machine learning to improve the efficiency of medical 3D printing by automating the image segmentation process, enhancing image acquisition, and facilitating the selection of the most appropriate subjects for 3D printing (Expert Rev Med Devices, Vol. 15:5, pp. 349-356).
New machine learning-based tools will likely help alleviate the burden of simple tasks that radiologists do on a daily basis, such as measuring anatomical structures and calculating volumes, Trevor Huff, first author and a third-year medical student at Creighton University School of Medicine, told AuntMinnie.com.
"If the demand for 3D-printed models in the hospital setting continues to grow, then there will likely need to be software capable of keeping up with the demand since image segmentation is arguably the most time-consuming part of the entire process," he said.
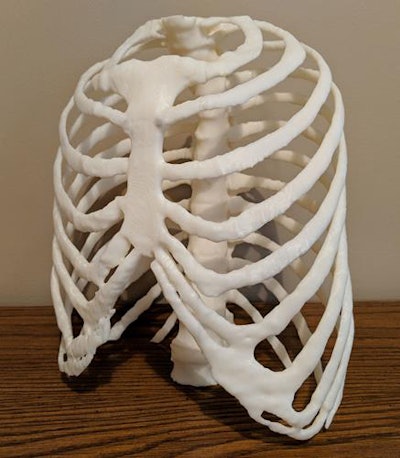
Overcoming challenges
Though a growing a number of studies have demonstrated the advantages of using 3D-printed models in healthcare for training, presurgical planning, and patient education, the integration of 3D printing into healthcare continues to be limited by issues tied to cost and production.
The amount of capital required to start and maintain a 3D printing lab may be beyond the means of many healthcare institutions, according to the authors. In addition to the cost of 3D printing machines and models, 3D printing labs also need to account for the cost of software packages, which are valued at an average of $32,025.
The lengthy process for creating 3D-printed models is yet another barrier to the implementation of 3D printing in healthcare, they noted. Producing a model often entails an extensive procedure involving patient selection, image acquisition, image segmentation, and printing, all of which have their own time constraints.
In line with the rising popularity of AI, proponents of medical 3D printing including Huff and colleagues have been utilizing advances in machine learning to overcome some of these challenges.
Multiple studies have shown that sufficiently trained machine-learning algorithms could potentially improve the efficiency of medical 3D printing in a variety of ways, such as by accomplishing the following:
- Streamlining patient selection and scheduling
- Decreasing scan times without sacrificing image quality
- Minimizing exposure to ionizing radiation
- Improving the quality of MRI and CT scans
- Cutting 3D printing processing times
- Reducing the need for trained segmentation specialists
By automating steps involved in 3D printing, machine-learning algorithms offer the potential to both increase processing speed and decrease dependence on technical specialists -- ultimately lowering costs, according to the authors.
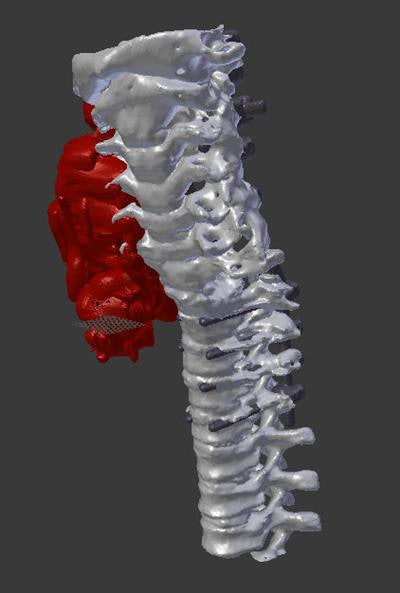
Segmenting images
In terms of increasing the efficiency of generating 3D-printed models, machine-learning algorithms may afford the greatest improvement to image segmentation, Huff said. Advancements in this area in the past few years have made quick medical image segmentation a possibility.
"We decided to work on the problems we were facing from all different angles, which included finding and possibly developing software solutions for the time-consuming process of image segmentation," he said. "This is where our main focus on machine learning has been in our efforts so far."
Currently, a specialist in 3D printing processes is responsible for any steps related to image segmentation and 3D modeling, the authors noted. As such, the quality of the finished product varies based on the skill of the specialist. And the turnaround time is often capped due to the limited availability of trained specialists.
Rather than use expensive proprietary software to process images for 3D printing, several groups have been using machine-learning algorithms to automate the segmentation of medical images. Early efforts in the medical community, including those by Akkus et al and Liu et al, have demonstrated that automated image segmentation can outperform standard methods, especially in musculoskeletal and brain segmentations.
Clinicians may soon be able to turn to open-source, automated image segmentation services and eliminate the need to pay for segmentation software and specialists, according to the researchers. They pointed to the deep-learning engine of big-data visualization software developer Zebra Medical Vision as a testament to machine learning's ability to provide low-cost image analysis.
Working together
The researchers acknowledged a major limitation of applying machine-learning algorithms to medical image segmentation and 3D printing in general: the lack of accessible data.
Collecting and testing large amounts of data is a "collaborative effort, and radiologists are essential to making something like this successful in clinical practice," Huff said. "They are the ones who will be using these solutions in clinical practice and therefore should be closely involved in all forms of development."
More recently, Huff and colleagues have been preparing the launch of a machine-learning research lab at the University of Nebraska Medical Center in collaboration with Dr. Justin Cramer, a neuroradiologist at the center. Along with a collection of graduate students, radiologists, surgeons, and imaging technologists from several institutions, they are designing software that they hope will be able to automatically segment brain structures on medical images.
"There seems to be an abundance these days of computer scientists interested in medical software development and radiologists who are interested in machine learning," Huff said. "Finding ways to work together on these types of projects is extremely important."