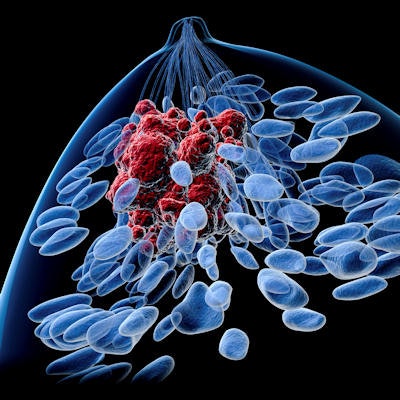
Artificial intelligence (AI) algorithms that utilize 4D information from dynamic contrast-enhanced MRI (DCE-MRI) can be highly accurate for classifying breast lesions, according to research published February 24 in Radiology: Artificial Intelligence.
A team of researchers led by Qiyuan Hu of the University of Chicago developed a new methodology to incorporate 4D volumetric and temporal information from DCE-MRI into 2D deep-learning analysis of breast lesions. They found that their approach, which utilizes a maximum intensity projection (MIP) of lesion features extracted by a deep-learning algorithm from four dynamic time points in a DCE-MRI, yielded even better lesion classification performance than a prior method based on a MIP of only one postcontrast subtraction image.
"Incorporating 4D information in DCE-MRI by feature MIP in deep transfer learning demonstrated superior classification performance compared with using MIP images as input in the task of distinguishing between benign and malignant breast lesions," the authors wrote.
Researchers from the University of Chicago had previously developed a method to make use of 4D information available in DCE-MRI, while also benefiting from the efficiency of utilizing pretrained 2D convolutional neural networks (CNNs) in developing computer-aided diagnosis (CADx) algorithms. That method, which they call image MIP, "collapsed" the 4D DCE-MRI lesion regions of interest from second postcontrast subtraction images into 2D MIPs. In testing, the image MIP approach outperformed other methods based only on 2D or 3D information.
Believing that they could achieve even better results, the researchers created a new technique for this study that they believe more effectively incorporates DCE-MRI's volumetric and temporal information.
"Instead of collapsing the volumetric information at the image level to form MIP images, we do so at the feature level by taking the maximum of CNN features along the axial dimension for a given lesion directly within the deep neural network architecture," they wrote.
This approach, which they call "feature MIP," also captures richer temporal information by including subtraction images from four dynamic points in a DCE sequence, according to the researchers. These subtraction images are input into the red, green, and blue channels of a CNN.
"Since feature MIP may more effectively leverage the volumetric information in DCE-MRI, we hypothesized that the deep learning with feature MIP would achieve higher classification performance than deep learning with image MIP in the task of distinguishing between benign and malignant breast lesions," the authors wrote.
Using a dataset of 1,455 lesions, the researchers trained linear support vector machine (SVM) classifiers to characterize lesions using features extracted from the CNNs based on either the image MIP or feature MIP approach. The feature MIP method yielded a significantly improved performance on an independent test set of 535 lesions.
Performance of feature MIP for breast lesion characterization | ||
Algorithm based on image MIP method | Algorithm based on feature MIP method | |
Area under the curve | 0.91 | 0.93* |
In the future, the researchers plan to extend the analysis to include other T2-weighted and diffusion-weighted MRI sequences in multiparametric MRI.
"We will also expand the database to include images from different medical centers and populations to evaluate the robustness of our method across imaging manufacturers, facility protocols, and patient populations," the authors wrote.