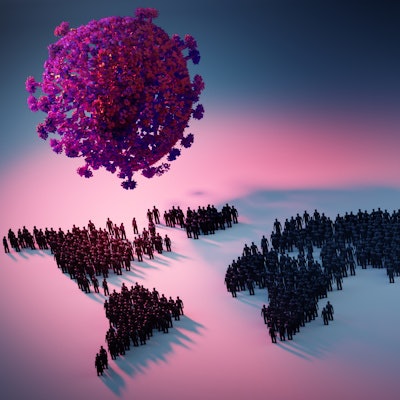
Radiomics features on noncontrast-enhanced chest CT exams can help to identify which patients with COVID-19 pneumonia will need to be admitted to the intensive care unit (ICU). They can also predict patient outcomes, according to research published online July 23 in Radiology: Cardiothoracic Imaging.
A research team led by first author Dr. Fatemeh Homayounieh and Dr. Mannudeep Kalra of Harvard Medical School in Boston evaluated the use of radiomics in noncontrast-enhanced chest CT scans from over 300 patients with COVID-19 pneumonia from a teaching hospital in Iran. They found that radiomics yielded better predictions than radiologist interpretations for predicting patient outcomes, disease severity, and triage.
"Along with the clinical variables, whole-lung radiomics can be a powerful tool for assessing diffuse pulmonary parenchymal diseases such as the viral pneumonia assessed in our study," the authors wrote. "Such prediction can help in allocation and planning of resources in high-prevalence diseases such as the current COVID-19 pandemic."
Chest CT is frequently used to diagnose and assess COVID-19 pneumonia in resource-starved locations that have limited access to reverse transcription polymerase chain reaction (RT-PCR) assays, as well as in high-risk patients with negative initial RT-PCR results, according to the authors. The researchers utilized a sample of 315 adult patients from a teaching hospital in Iran to compare the performance of whole-lung radiomics, the interpretations of radiologists, and clinical variables in predicting disease outcome, severity, and patient triage in cases of COVID-19 pneumonia.
The patients included 240 inpatients and 120 outpatients, and all received CT scans on a Somatom Emotion 16 16-slice CT scanner (Siemens Healthineers). Two radiologists assessed each CT exam in consensus and graded the extent of pulmonary involvement and type of opacities within each lobe.
Using the CT scan's 2-mm images, both lungs were segmented semiautomatically using the open-source Radiomics 3D Slicer Extension program. Next, a total of 1,690 radiomics features were extracted from the entire lung volumes, including both the abnormal and normal lung parenchyma.
The researchers found that the best-performing radiomics features were superior to the subjective assessment of radiologists in predicting patient outcome and severity of COVID-19 pneumonia. They also found that adding clinical variables and laboratory data slightly improved both outcome prediction and the need for patients to be admitted to the ICU.
Radiomics vs. radiologists for predicting results in patients with COVID-19 pneumonia, by area under the curve | |||
Radiologist assessment | Radiomics | Radiomics + clinical variables | |
Patient triage (inpatient vs. outpatient) | 0.69 | 0.84 | n/a |
Need for ICU admission among inpatients | 0.68 | 0.77 | 0.84 |
Patient outcome | 0.68 | 0.81 | 0.85 |
The researchers believe that their study provides evidence that whole-lung radiomics information should be integrated into radiology reports. The capability to automatically segment the whole lung -- available via both open-source and commercial image processing platforms -- can avoid or minimize the effort required by radiologists to obtain radiomics information, according to the authors.
"However, institutions would require datasets such as in our study to establish a training model so that individual prospective cases can be tested against such local models to account for local variations in CT scanners, scan parameters and patient characteristics," they wrote. "For such integration [of radiomics] to happen, both the radiologists and referring physicians need to understand the principles, strengths and limitations of radiomics so that meaningful inferences can be drawn from a large amount of quantitative information generated from radiomics."