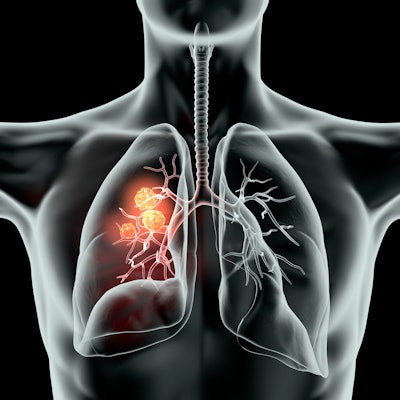
CT radiomics can be useful for predicting survival in patients receiving immunotherapy for non-small cell lung cancer (NSCLC), according to research presented at this week's annual meeting of the American Association for Cancer Research (AACR).
A specific CT radiomics feature was associated with survival in seven different patient cohorts, according to presenter Robert Gillies, PhD, of the H. Lee Moffitt Cancer Center and Research Institute in Tampa, FL
"[The CT radiomics] feature is a pansignature for overall survival," he said.
PD-L1 expression
Traditionally determined via immunohistochemistry, expression of programmed cell death ligand 1 (PD-L1) has been shown to be an indicator for utilizing checkpoint inhibitor treatments such as pembrolizumab. However, PD-L1 expression can be heterogeneous, leading to subsampling artifacts, according to Gillies. And it's also time-variant.
"So an improved biomarker to predict response would be warranted," he said.
A team of researchers led by Ilke Tunali, PhD, sought to test its hypothesis that quantitative imaging could identify PD-L1 status and predict treatment response. The group first gathered a cohort of 325 patients with NSCLC who received anti-PD-1 therapy. These patients were separated into a training cohort of 180 patients and a validation cohort of 90 patients from Moffitt Cancer Center, as well as an external test cohort of 55 patients from the Veterans Affairs Hospital in Tampa.
The cohort included a variety of treatment responders. Of the first 228 patients in the cohort, 74 were nonresponders (< 3 months), 93 were short-term responders (> 3 months and < 12 months), 20 were long-term responders (> 12 months), and 41 were ongoing responders (> 12 months).
Clinical, radiomics variables
The researchers analyzed both clinical variables and radiomics features to build a parsimonious model for categorizing patients into risk groups. They winnowed an initial group of 11 patient characteristics and five hematology results down to two significant clinical variables: serum albumin and the number of metastases.
After next extracting over 600 radiomics features, Tunali and colleagues found that 13 were associated with overall survival. Those features were highly correlated, however, and one radiomics measure of tumor heterogeneity -- gray-level co-occurrence matrix (GLCM) inverse difference -- was chosen to represent the group of features and serve as what the researchers call an "avatar" feature.
Along with serum albumin results and the number of metastatic sites, the avatar radiomics feature was used to create a decision tree based on defined thresholds. This classification and regression tree (CART) categorizes patients into risk groups based on defined thresholds of the GLCM inverse difference, serum albumin, and the number of metastatic sites.
Performance for this CART risk prediction analysis was then assessed on both the training and validation cohort, as well as the external patient cohort.
Performance of radiomics/clinical model for predicting survival in NSCLC patients | ||
Training and validation cohort | External patient cohort | |
Low-risk group | Hazard ratio (HR) = 1 | HR = 1 |
Moderate-risk group | HR = 3.3 | HR = 1.7 |
High-risk group | HR = 9.2 | HR = 2.7 |
Very high-risk group | HR = 19.2 | HR = 10.5 |
To determine if their approach is predictive specifically of immunotherapy or if it's generally prognostic for patient outcomes, the researchers applied their decision tree to four previously curated cohorts of patients. These cohorts included 62 adenocarcinoma cases that were treated with targeted therapy, 102 cases that included gene expression data and were treated with chemotherapy, 47 NSCLC patients treated with radiotherapy from the Maastro Clinic in the Netherlands, and 226 patients from the National Lung Screening Trial.
A panprognostic feature
The combination of the radiomics feature and the two clinical features was able to separate responders and nonresponders in all four of these cohorts, according to Gillies.
"So we think this gray-level co-occurrence feature is a panprognostic feature for [predicting] outcome," he said.
After conducting further analysis of the gene expression cohort in an effort to understand the biological underpinnings of the radiomics feature, the researchers found a high correlation with carbonic anhydrase 9 (CA9) mRNA expression. This association was also confirmed on immunohistochemistry analysis.
"It's positively associated with carbonic anhydrase 9, which is upregulated in hypoxic tumors," Gillies said. "And hypoxic tumors are known to be more resistant to therapy response."
He also shared results of related radiomics work conducted at Moffitt Cancer Center that was previously presented in January at the AACR Virtual Special Conference on Artificial Intelligence, Diagnosis, and Imaging. In that study, Wei Mu, PhD, and colleagues reported that the combination of PET/CT radiomics and artificial intelligence could be used to guide treatment decisions for patients with advanced NSCLC.