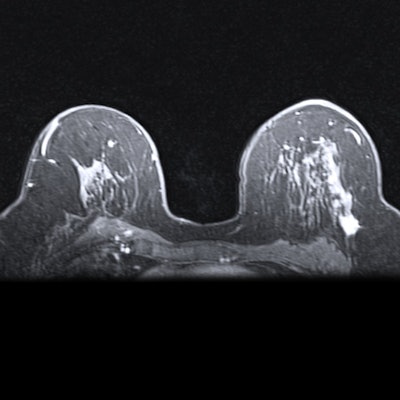
Artificial intelligence (AI) models trained on radiomics and clinical imaging findings can accurately detect cancer on breast MRI, according to a May 17 talk at the annual International Society for Magnetic Resonance in Medicine (ISMRM) meeting.
South Korean researchers trained machine-learning algorithms based on MRI radiomics features alone and then in combination with specific clinical imaging findings reported by radiologists. In testing, they found that the fusion models best differentiated new lesions identified on multiparametric breast MRI exams in breast cancer patients.
"Our results suggest that this approach may provide a noninvasive imaging tool to guide proper management that can reduce the use of unnecessary ultrasound or biopsy," said presenter Tien Anh Nguyen from Chonnam National University in Gwangju.
MRI is widely used to preoperatively assess the extent of breast cancer and to detect additional lesions that hadn't been identified on prior mammograms or ultrasound exams, according to Nguyen.
"It is very important to accurately predict malignancy of any additional MR-detected lesions before surgery due to potentially different treatment options," he said.
The researchers sought to investigate whether machine-learning algorithms combining radiomics and clinical imaging analysis could predict malignancy in additional enhancing lesions spotted on multiparametric breast MRI. They trained and tested algorithms using a dataset of 174 patients with primary breast cancer and who were found to have additional enhancing lesions on preoperative 3-tesla MRI. All of the additional lesions were pathologically confirmed to be malignant or benign.
Of the 174 patients, 80% were used for training and 20% were set aside for testing a variety of models. The researchers used least absolute shrinkage and selection operator regression analysis to select the five best radiomics features for both T1-weighted subtraction images and T2-weighted images.
They then utilized support vector machine (SVM) classifiers for training models based on either T1 subtraction images or T2 images. A model based only on six specific clinical imaging employed a decision tree, while fusion radiomics and clinical imaging models used SVM classifiers.
In testing, the fusion models yielded higher accuracy than the clinical imaging decision-tree model or the models based just on radiomics features.
Performance of machine-learning models for distinguishing malignant breast tumors | |||||
Decision tree model based on clinical imaging features | Model based on T2 radiomics features | Model based on T1 subtraction radiomics features | Fusion model combining radiomics of T1 subtraction images and clinical imaging features | Model combining T2 radiomics and clinical imaging features | |
Sensitivity | 61% | 73% | 94% | 83.3% | 94.1% |
Specificity | 82% | 67% | 59% | 76.5% | 75% |
Accuracy | 71% | 70% | 77% | 80% | 86.2% |
Area under the curve | 0.73 | 0.75 | 0.76 | 0.889 | 0.926 |
"In this study, we have proposed an effective method for extracting radiomics features from breast MRI and demonstrated that machine-learning methods combining features from radiomics and clinical imaging interpretation using multiparametric MRI could be used to predict malignancy in additional MR-detected lesions in patients with breast cancer," Nguyen said.