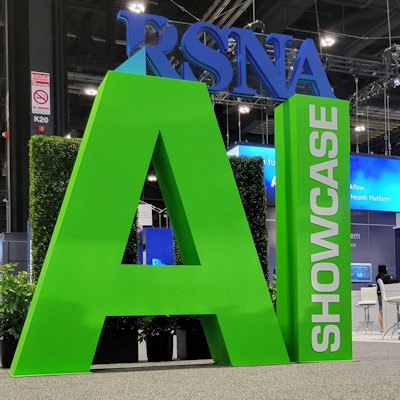
An artificial intelligence (AI) algorithm was able to enable spine MRI exams to be acquired as much as 72% faster while improving image quality, according to a presentation Thursday at RSNA 2021 in Chicago.
Researchers led by presenter Dr. Lawrence Tanenbaum of imaging services provider RadNet compared the performance of a commercial deep-learning algorithm with traditional methods for accelerating spine MRI in clinical practice. In a study involving 26 patients, two neuroradiologists overwhelmingly preferred the image quality of the AI-reconstructed images to those acquired by standard-of-care methods that required much longer scanning time.
"Deep-learning reconstruction provides gains in efficiency and improvement in the patient experience, portending the utility in routine clinical practice," Tanenbaum said.
In their study, the researchers sought to test their hypothesis that data acquired with an accelerated acquisition and reconstructed with a deep-learning method could yield comparable image quality to traditional, longer acquisition techniques.
"We've been using deep learning for a while and we essentially went back and said, 'Let's look at the data acquired from an accelerated acquisition with a deep-learning reconstruction against the best approximation of [what we would produce in] clinical practice with a traditional technique,' " he said.
The study authors assessed the impact of the Air Recon DL image reconstruction software (GE Healthcare) on image quality and scan times in 26 consecutive patients receiving cervical and lumbar spine MRI. The k-space-based tool provides denoising, reductions of artifacts (such as truncation ringing), sharpness enhancement, and improved contrast-to-noise and signal-to-noise ratios.
The patients underwent both standard-of-care and either accelerated cervical (11 cases) or lumbar (15 cases) spine MRI on an Architect 3-tesla scanner (GE). The accelerated acquisitions were 72% faster for cervical scans and 62% faster for lumbar scans.
Sagittal T2 images were essentially acquired twice, once with the local standard-of-care spine parameters and another using an accelerated protocol. The accelerated scan data was then processed using the AIR Recon DL software.
Two neuroradiologists then evaluated the different image series paired in side-by-side datasets, which were blinded and randomized in sequence and also left-to-right display order. Using a five-point Likert scale, the readers assessed the images for overall image quality, anatomy conspicuity, cord/cerebrospinal fluid/lesion contrast, sharpness, apparent signal-to-noise ratio, artifacts, and aberrations.
Across all six categories, both neuroradiologists strongly preferred the deep learning-reconstructed images, particularly in overall image quality.
Impact of AI-based spine MRI reconstruction on radiologist preferences | |||
Strong preference for deep-learning images | Preference for deep-learning images | No preference or preference for standard-of-care images | |
Overall image quality of cervical scans | 77.3% | 22.7% | 0% |
Overall image quality for lumbar scans | 76.7% | 23.3% | 0% |
What's more, the readers had a mean Likert score difference of only -0.26, demonstrating very high interrater agreement, according to the authors.
"The deep learning-processed images were strongly favored over the images acquired from the longer protocol, which attempted to approximate the resolution of the deep-learning protocol," Tanenbaum said.