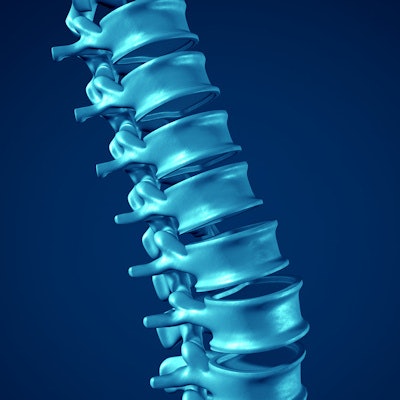
A team of Chinese researchers has developed an artificial intelligence (AI) algorithm that creates x-ray-like images from ultrasound data. They describe how they are using the technique to visualize adolescent idiopathic scoliosis in a study published July 29 in Ultrasonics.
The results could be good news for children at risk of scoliosis progression as their bodies grow, a condition known as adolescent idiopathic scoliosis (AIS) that affects 1% to 3% of the adolescent population. The technique could reduce their radiation exposure and thus perhaps risk of future cancer, wrote a team led by Weiwei Jiang, PhD, of Zhejiang University of Technology in Hangzhou, China.
"Monitoring of curve progression typically involves routine x-ray radiography throughout childhood and adolescence," the investigators noted. "However, cumulative radiation exposure ... has been reported to increase cancer risk."
Scoliosis is diagnosed by using x-ray of standing patients to measure the distance between a straight line and a scoliotic curve in the spine (i.e., Cobb's method to measure Cobb's angle).
But the radiation it imparts raises concerns about repeated use, especially in an adolescent population, the group wrote.
Ultrasound, on the other hand, doesn't use radiation and can deliver images in real-time. It has also been used to evaluate scoliosis by combining the 2D probe with 3D position sensors to produce 3D ultrasound data. But this method is time-consuming and doesn't produce a full image of the spine.
"Ultrasound imaging methods ... are nonionizing and real-time, making them potentially useful for AIS screening, progress monitoring, and treatment outcome evaluation," the authors explained. "However, because ultrasound cannot penetrate bony structure, it uses surface reflections for imaging and cannot provide bony details."
To address this conundrum, Jiang's team developed an algorithm called UXGAN (Ultrasound to X-ray Generative Attentional Network) that creates synthesized x-ray-like images from ultrasound data. In a study that included 202 children with adolescent idiopathic scoliosis -- all of whom underwent both ultrasound and x-ray -- the group compared Cobb angle values generated on the synthesized x-ray images to actual x-rays. The team also compared UXGAN's performance to three other algorithms (based on an AI training technique called CycleGAN).
The investigators found that the synthesized x-ray image of the spine was comparable in terms of imaging the structure and curvature of the spine as the actual x-ray image: For example, the Cobb angle for one of the generated x-rays was10.3 degrees, and for the real x-ray, 10 degrees. UXGAN also outperformed the other CycleCAN algorithms across the following measures:
- Normalized mean squared error (NMSE), which measures pixel intensity distance*
- Peak signal-to-noise ratio (PSNR), which evaluates synthesis accuracy
- Structural similarity (SSIM), which represents the structural similarity between the ultrasound and synthesized x-ray images
*(lower NMSE values translate to higher PSNR and SSIM values and thus better image quality)
The study findings show significant promise, according to Jiang and colleagues.
"[Our] experimental results show the reliability and accuracy of the method," they concluded. "This method can provide radiation-free ultrasound and x-ray images, which is of great significance for diagnosing and monitoring adolescent idiopathic scoliosis."