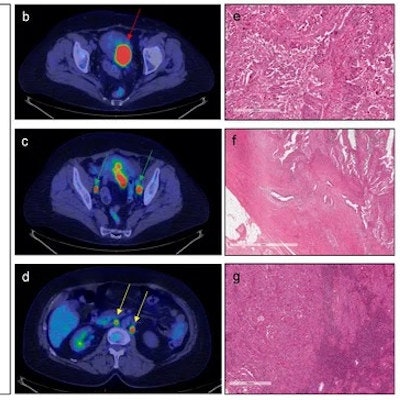
A machine-learning model based on PET imaging features shows promise for helping clinicians assess the aggressiveness of endometrial cancer prior to surgery, according to a study published online January 4 in Cancers.
An Italian group at Vita-Salute San Raffaele University in Milan developed an artificial intelligence (AI) algorithm based on standard F-18 FDG-PET imaging features and clinical data of primary tumors in patients with endometrial cancer. The model performed well in testing and could be useful for supporting preoperative treatment management of these patients, they wrote.
"The present work reports one of the first analyses evaluating the role of machine learning-based classification using F-18 FDG-PET-derived parameters in predicting features of [endometrial cancer] aggressiveness, which are currently assessable only after surgery," the group wrote.
Endometrial cancer is a type of cancer that begins in the uterus. Early and accurate assessment of the cancer is crucial for correct treatment planning in patients. Radical surgery and lymphadenectomy are generally recommended for high-risk patients.
![A 71-year-old patient with endometrial cancer who underwent F-18 FDG-PET/CT for staging purposes. Red arrows indicate pathological uptake in correspondence of the primary tumor. Green arrows indicate pathological uptake in correspondence of bilateral iliac-obturator lymph nodes, and yellow arrows indicate pathological uptake in correspondence of lomboaortic and interaortocaval lymph nodes [(a): Maximum intensity projection; (b–d): transaxial PET/CT images]. PET parameters of the primary tumor were as follows: SUVmax = 17.43; SUVmean = 11.82; MTV = 28.60; TLG = 338.04. Histological analysis: the tumor is constituted by large cells with a high grade of nuclear atypia and numerous mitotic figures (e). The tumoral growth is mainly in papillary projections. Myometrial infiltration (f) has a tubulo-glandular architecture with micropapillary structure into the lumen; the way of myometrial invasion is infiltrative/destructive. Lymph nodal metastasis (g) is nodular and constituted by serous atypical cells arranged in cords and small nests. Image and caption courtesy of Cancers and licensed under CC BY 4.0.](https://img.auntminnie.com/files/base/smg/all/image/2023/01/am.2023_01_09_21_32_2698_2023_01_09_AI_endometrial_cancer.png?auto=format%2Ccompress&fit=max&q=70&w=400)
Conventional imaging with transvaginal ultrasound, MRI, and CT provides detailed morphological information but only limited assessment of functional characteristics. Moreover, biopsy may not represent the whole tumor's heterogeneity and thus may provide only limited information on tumor aggressiveness before surgery, the authors wrote.
In this study, the researchers developed a machine-learning model based on parameters indicating tumor aggressiveness from F-18 FDG-PET/CT or F-18 PET/MRI scans in 123 patients who underwent imaging for surgery staging purposes between August 2009 and February 2021. Seventy-six patients were classified as high-intermediate/high risk.
PET parameters included maximum standardized uptake value (SUVmax), SUVmean, metabolic tumor volume (MTV), and total lesion glycolysis (TLG). Together and individually, these parameters were compared to clinical metrics of tumor aggressiveness, such as histotype, myometrial invasion (MI), risk group, lymph node involvement (LN), and expression of the p53 gene.
The model predicted MI with an accuracy of 87% using all PET parameters and age; the patients' risk group was predicted with an accuracy of 79% using all PET parameters. In addition, LN involvement was predicted with an accuracy of 83% using MTV and TLG. Finally, p53 expression was predicted with an accuracy of 73% using SUVmax and SUVmean, according to the findings.
"The present study demonstrates the valuable role of [machine learning]-based classification using conventional F-18 FDG-PET parameters and clinical data in predicting features of tumor aggressiveness in [endometrial cancer] patients," the authors wrote.
The authors noted that one strength of the proposed model is that it analyzes conventional PET semiquantitative imaging data (combined with clinical data) in the primary staging of endometrial cancer patients compared to radiomics features, which are more difficult to extract.
"This is particularly significant for both a better physician interpretability of the model's outcomes and translation into the clinical practice," the authors concluded.