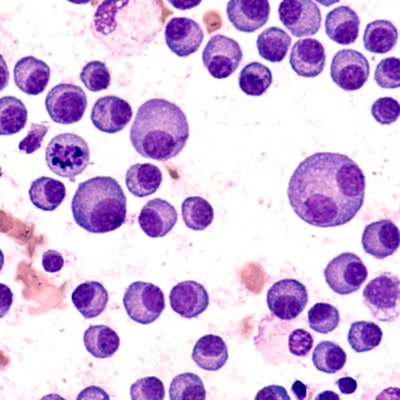
Radiomics models based on F-18 FDG-PET/CT imaging features and powered by machine learning can improve prediction for outcomes in multiple myeloma patients, according to research published June 27 in BMC Medical Imaging.
A team led by Haoshin Zhong from the Affiliated Hospital of Southwest Medical University in Luzhou City, Sichuan, China, found that their model achieved high-performance marks in the prognosis of multiple myeloma.
"F-18 FDG PET/CT based radiomics models ... can significantly improve the clinical prediction of progress and increased clinical benefits providing prospects for clinical prognostic stratification for precision treatment as well as new research areas," Zhong and colleagues wrote.
While multiple myeloma prognosis has been studied through clinical parameters, there's been a lack of data on how dual imaging modalities and multiple algorithms can further improve prognosis estimation in patients, according to the researchers. Using F-18 FDG-PET/CT-based radiomics, the Zhong team used data from 98 multiple myeloma patients to develop and test a number of models for predicting multiple myeloma prognosis.
The models incorporated different combinations of PET, CT, clinical parameters, and one of six types of machine-learning algorithms: Cox, gradient boosted-Cox, CoxBoost, a generalized boosted model, random forest survival, and support vector classification regression.
The final models all utilized five PET-based features, four CT-based features, and six clinically derived features that were all significantly related to progression-free survival. Following this, the team validated the resulting radiomics models using the bootstrap method for repeated resampling of data samples to generate larger sample sets.
The researchers found that the model that combined the generalized boosted algorithm, clinical parameters, and CT-based radiomics had the best performance on the training set with an average AUC of 0.961. However, for the validation group, the best model was the combined model of the random forest survival algorithm, clinical parameters, and PET-based radiomics features. This model yielded an average AUC of 0.88.
The researchers also found via time-dependent receiver operating characteristic (ROC) analysis that for each algorithm, the addition of PET or CT features to clinical parameters improved the prediction prognosis during the follow-up period.
The study authors suggested that based on their findings, the radiomics signature is "critical" when it comes to patient prognosis. They wrote that this provides prognostic efficiency of bone marrow PET/CT radiomics in patients, as well as provides clinical risk stratification.
"This report offers an essential supplementary reference for radiomics-based prognostic models as we compared numerous machine learning methods," the authors wrote. "This large-scale comparison is beneficial for the accurate selection of machine learning methods for radiomics-based progression free survival estimation."
The researchers recommend that their proposed prognostic model now be evaluated on a large, multicenter patient population and validated on an external, independent validation cohort.
The study can be found here.